Influence of high Andean grasslands on landslide reduction in Peru
DOI:
https://doi.org/10.17268/sci.agropecu.2024.025Palavras-chave:
High Andean grasslands, landslide, machine learning, ecosystem services, climate changeResumo
Agricultural and urban expansion has caused considerable degradation of ecosystems. In the case of Peruvian high Andean grasslands, it was reported that between 2000 and 2009, this ecosystem was reduced by 7%. The limited or no protection they receive is partly due to the fact that the benefits of ecosystem services are not widely known. This research aims to establish and predict the influence of high Andean grasslands on the annual occurrence of landslides. To do so, we identified occurrences of landslides, falls, huaycos, avalanches, and alluviums in high Andean grasslands. We also examined urban areas and agricultural zones of Peru for the period from 2003 to 2016. Subsequently, we extracted data on precipitation, temperature, slopes, soil types, and geographical variables. This data was used to train a machine learning model. The results show that 96% of landslides occurred in human-intervened areas, and only 4% in high Andean grasslands. Precipitation and slope thresholds for landslide occurrence are higher in high Andean grasslands compared to agricultural and urban areas. The best-performing machine learning models were linear regression, Gaussian processes, random forest, and support vector machine. They had coefficients of determination of R² = 0.80, 0.80, 0.66, and 0.64, respectively. Predictions show that if agricultural or urban areas are established in wet or dry puna grasslands, the average number of occurrences multiplies. The multiplier factors are 2.1 and 7.08, the number of deaths by 2.8 and 10.49, the number of houses destroyed by 2.4 and 7.51, and the number of roads destroyed by 2.2 and 7.37, respectively. The study demonstrates that conserving high Andean grasslands significantly reduces landslides compared to urban or agricultural areas.
Referências
Adnan, M. S. G., Rahman, M. S., Ahmed, N., Ahmed, B., Rabbi, Md. F., & Rahman, R. M. (2020). Improving Spatial Agreement in Machine Learning-Based Landslide Susceptibility Mapping. Remote Sensing, 12(20). https://doi.org/10.3390/rs12203347
Alavi-Murillo, G., Diels, J., Gilles, J., & Willems, P. (2022). Soil organic carbon in Andean high-mountain ecosystems: importance, challenges, and opportunities for carbon sequestration. Regional Environmental Change, 22(4), 128. https://doi.org/10.1007/s10113-022-01980-6
Al-Najjar, H. A. H., & Pradhan, B. (2021). Spatial landslide susceptibility assessment using machine learning techniques assisted by additional data created with generative adversarial networks. Geoscience Frontiers, 12(2), 625–637. https://doi.org/10.1016/J.GSF.2020.09.002
Arabameri, A., Chandra Pal, S., Rezaie, F., Chakrabortty, R., Saha, A., Blaschke, T., Di Napoli, M., Ghorbanzadeh, O., & Thi Ngo, P. T. (2022). Decision tree based ensemble machine learning approaches for landslide susceptibility mapping. Geocarto International, 37(16), 4594–4627. https://doi.org/10.1080/10106049.2021.1892210
Aybar, C., Fernández, C., Huerta, A., Lavado, W., Vega, F., & Felipe-Obando, O. (2020). Construction of a high-resolution gridded rainfall dataset for Peru from 1981 to the present day. Hydrological Sciences Journal, 65(5), 770–785. https://doi.org/10.1080/02626667.2019.1649411
Basu, T., Das, A., & Swades, P. (2022). Application of geographically weighted principal component analysis and fuzzy approach for unsupervised landslide susceptibility mapping on Gish River Basin, India. Geocarto International, 37(5), 1294–1317. https://doi.org/10.1080/10106049.2020.1778105
Bonnesoeur, V., Locatelli, B., Guariguata, M. R., Ochoa-Tocachi, B. F., Vanacker, V., Mao, Z., Stokes, A., & Mathez-Stiefel, S. L. (2019a). Impacts of forests and forestation on hydrological services in the Andes: A systematic review. Forest Ecology and Management, 433, 569–584. https://doi.org/10.1016/J.FORECO.2018.11.033
Bonnesoeur, V., Locatelli, B., Guariguata, M. R., Ochoa-Tocachi, B. F., Vanacker, V., Mao, Z., Stokes, A., & Mathez-Stiefel, S. L. (2019b). Impacts of forests and forestation on hydrological services in the Andes: A systematic review. Forest Ecology and Management, 433, 569–584. https://doi.org/10.1016/J.FORECO.2018.11.033
Borgatti, L., & Soldati, M. (2018). The influence of Holocene climatic changes on landslide occurrence in Europe. In Landslides (1st ed., pp. 111–116). Routledge. https://doi.org/10.1201/9780203749197-8
Brenning, A.: Spatial prediction models for landslide hazards: review, comparison and evaluation. Nat. Hazards Earth Syst. Sci., 5, 853–862, https://doi.org/10.5194/nhess-5-853-2005, 2005.
Bui, D. T., Shahabi, H., Omidvar, E., Shirzadi, A., Geertsema, M., et al. (2019). Shallow Landslide Prediction Using a Novel Hybrid Functional Machine Learning Algorithm. Remote Sensing, 11(8), 931. https://doi.org/10.3390/RS11080931
Bui, D. T., Tsangaratos, P., Nguyen, V. T., Liem, N. Van, & Trinh, P. T. (2020). Comparing the prediction performance of a Deep Learning Neural Network model with conventional machine learning models in landslide susceptibility assessment. CATENA, 188, 104426. https://doi.org/10.1016/J.CATENA.2019.104426
Cerri, R. I., Reis, F. A. G. V., Gramani, M. F., Giordano, L. C., & Zaine, J. E. (2017). Landslides Zonation Hazard: relation between geological structures and landslides occurrence in hilly tropical regions of Brazil. Anais Da Academia Brasileira de Ciências, 89(4), 2609–2623. https://doi.org/10.1590/0001-3765201720170224
Chang, Z., Huang, J., Huang, F., Bhuyan, K., Meena, S. R., & Catani, F. (2023). Uncertainty analysis of non-landslide sample selection in landslide susceptibility prediction using slope unit-based machine learning models. Gondwana Research, 117, 307–320. https://doi.org/https://doi.org/10.1016/j.gr.2023.02.007
Chen, C. Y., & Huang, W. L. (2013). Land use change and landslide characteristics analysis for community-based disaster mitigation. Environmental Monitoring and Assessment, 185(5), 4125–4139. https://doi.org/10.1007/S10661-012-2855-Y/METRICS
Chen, L., Guo, Z., Yin, K., Pikha Shrestha, D., & Jin, S. (2019). The influence of land use and land cover change on landslide susceptibility: A case study in Zhushan Town, Xuan’en County (Hubei, China). Natural Hazards and Earth System Sciences, 19(10), 2207–2228. https://doi.org/10.5194/NHESS-19-2207-2019
Cossios-Meza, E. D. (2018). Informe sobre estado y tendencias de la diversidad de ecosistemas del Perú. https://cdn.www.gob.pe/uploads/document/file/321470/Estado_y_tendencias_ecosistemas.pdf
Dahal, R. K., & Hasegawa, S. (2008). Representative rainfall thresholds for landslides in the Nepal Himalaya. Geomorphology, 100(3–4), 429–443. https://doi.org/10.1016/J.GEOMORPH.2008.01.014
Dai, A., Lin, X., & Hsu, K.-L. (2007). The frequency, intensity, and diurnal cycle of precipitation in surface and satellite observations over low- and mid-latitudes. Climate Dynamics 2007 29:7, 29(7), 727–744. https://doi.org/10.1007/S00382-007-0260-Y
Flores, E. (2016). Cambio Climático: Pastizales Altoandinos y Seguridad Alimentaria. Revista de Glaciares y Ecosistemas de Montaña, 1(1), 73–80. https://doi.org/10.36580/rgem.i1.73-80
Forte, G., Pirone, M., Santo, A., Nicotera, M. V., & Urciuoli, G. (2019). Triggering and predisposing factors for flow-like landslides in pyroclastic soils: the case study of the Lattari Mts. (southern Italy). Engineering Geology, 257, 105137. https://doi.org/10.1016/J.ENGGEO.2019.05.014
Glade, T. (2003). Landslide occurrence as a response to land use change: a review of evidence from New Zealand. CATENA, 51(3–4), 297–314. https://doi.org/10.1016/S0341-8162(02)00170-4
Goetz, J. N., Brenning, A., Petschko, H., & Leopold, P. (2015). Evaluating machine learning and statistical prediction techniques for landslide susceptibility modeling. Computers & Geosciences, 81, 1-11. https://doi.org/10.1016/j.cageo.2015.04.007.
Gupta, S. K., & Shukla, D. P. (2023). Handling data imbalance in machine learning based landslide susceptibility mapping: a case study of Mandakini River Basin, North-Western Himalayas. Landslides, 20(5), 933–949. https://doi.org/10.1007/s10346-022-01998-1
Hassangavyar, M. B., Damaneh, H. E., Pham, Q. B., Linh, N. T. T., Tiefenbacher, J., & Bach, Q.-V. (2022). Evaluation of re-sampling methods on performance of machine learning models to predict landslide susceptibility. Geocarto International, 37(10), 2772–2794. https://doi.org/10.1080/10106049.2020.1837257
Huang, F., Cao, Z., Guo, J., Jiang, S. H., Li, S., & Guo, Z. (2020). Comparisons of heuristic, general statistical and machine learning models for landslide susceptibility prediction and mapping. CATENA, 191, 104580. https://doi.org/10.1016/J.CATENA.2020.104580
Irigaray, C., Lamas, F., El Hamdouni, R., Fernández, T., & Chacón, J. (2000a). The importance of the precipitation and the susceptibility of the slopes for the triggering of landslides along the roads. Natural Hazards, 21(1), 65–81. https://doi.org/10.1023/A:1008126113789/METRICS
Irigaray, C., Lamas, F., El Hamdouni, R., Fernández, T., & Chacón, J. (2000b). The Importance of the Precipitation and the Susceptibility of the Slopes for the Triggering of Landslides Along the Roads. Natural Hazards 2000 21:1, 21(1), 65–81. https://doi.org/10.1023/A:1008126113789
Jiang, G., & Wang, W. (2017). Error estimation based on variance analysis of k-fold cross-validation. Pattern Recognition, 69, 94–106. https://doi.org/10.1016/J.PATCOG.2017.03.025
Kainthura, P., & Sharma, N. (2022). Hybrid machine learning approach for landslide prediction, Uttarakhand, India. Scientific Reports, 12(1), 20101. https://doi.org/10.1038/s41598-022-22814-9
Karsli, F., Atasoy, M., Yalcin, A., Reis, S., Demir, O., & Gokceoglu, C. (2009). Effects of land-use changes on landslides in a landslide-prone area (Ardesen, Rize, NE Turkey). Environmental Monitoring and Assessment, 156(1–4), 241–255. https://doi.org/10.1007/S10661-008-0481-5/METRICS
Khan, S., Kirschbaum, D. B., & Stanley, T. (2021). Investigating the potential of a global precipitation forecast to inform landslide prediction. Weather and Climate Extremes, 33, 100364. https://doi.org/10.1016/J.WACE.2021.100364
Klose, M., Auerbach, M., Herrmann, C., Kumerics, C., & Gratzki, A. (2017). Landslide Hazards and Climate Change Adaptation of Transport Infrastructures in Germany. Advancing Culture of Living with Landslides, 535–541. https://doi.org/10.1007/978-3-319-59469-9_48
Kuradusenge, M., Kumaran, S., & Zennaro, M. (2020). Rainfall-Induced Landslide Prediction Using Machine Learning Models: The Case of Ngororero District, Rwanda. International Journal of Environmental Research and Public Health 2020, Vol. 17, Page 4147, 17(11), 4147. https://doi.org/10.3390/IJERPH17114147
Liu, Z., Gilbert, G., Cepeda, J. M., Lysdahl, A. O. K., Piciullo, L., Hefre, H., & Lacasse, S. (2021). Modelling of shallow landslides with machine learning algorithms. Geoscience Frontiers, 12(1), 385–393. https://doi.org/10.1016/J.GSF.2020.04.014
Ma, Z., Mei, G., & Piccialli, F. (2020a). Machine learning for landslides prevention: a survey. Neural Computing and Applications 2020 33:17, 33(17), 10881–10907. https://doi.org/10.1007/S00521-020-05529-8
Ma, Z., Mei, G., & Piccialli, F. (2020b). Machine learning for landslides prevention: a survey. Neural Computing and Applications 2020 33:17, 33(17), 10881–10907. https://doi.org/10.1007/S00521-020-05529-8
Madrigal-Martínez, S., Miralles I García, J. L., Molina, L., & Lima, P. (2019). Land-change dynamics and ecosystem service trends across the central high-Andean Puna. Scientific Reports 2019 9:1, 9(1), 1–12. https://doi.org/10.1038/s41598-019-46205-9
Marc, O., Stumpf, A., Malet, J. P., Gosset, M., Uchida, T., & Chiang, S. H. (2018). Initial insights from a global database of rainfall-induced landslide inventories: The weak influence of slope and strong influence of total storm rainfall. Earth Surface Dynamics, 6(4), 903–922. https://doi.org/10.5194/ESURF-6-903-2018
Marin, R. J., & Velásquez, M. F. (2020). Influence of hydraulic properties on physically modelling slope stability and the definition of rainfall thresholds for shallow landslides. Geomorphology, 351, 106976. https://doi.org/10.1016/J.GEOMORPH.2019.106976
Meneses, B. M., Pereira, S., & Reis, E. (2019). Effects of different land use and land cover data on the landslide susceptibility zonation of road networks. Natural Hazards and Earth System Sciences, 19(3), 471–487. https://doi.org/10.5194/NHESS-19-471-2019
Merghadi, A., Abderrahmane, B., & Tien Bui, D. (2018). Landslide Susceptibility Assessment at Mila Basin (Algeria): A Comparative Assessment of Prediction Capability of Advanced Machine Learning Methods. ISPRS International Journal of Geo-Information 2018, Vol. 7, Page 268, 7(7), 268. https://doi.org/10.3390/IJGI7070268
Micheletti, N., Foresti, L., Robert, S., Leuenberger, M., Pedrazzini, A., Jaboyedoff, M., & Kanevski, M. (2013). Machine Learning Feature Selection Methods for Landslide Susceptibility Mapping. Mathematical Geosciences, 46(1), 33–57. https://doi.org/10.1007/S11004-013-9511-0
Mills, S. C., Parra Sanchez, E., Socolar, J. B., Bousfield, C., Coffey, B., Barlow, J., Quintero, J. M. O., Haugaasen, T., & Edwards, D. P. (2023). Avian biodiversity losses from grazing of high Andean páramo. Biological Conservation, 286, 110298. https://doi.org/https://doi.org/10.1016/j.biocon.2023.110298
MINAM. (2019a). Guía rápida del Programa Presupuestal 0144: Conservación y uso sostenible de ecosistemas para la provisión de servicios ecosistémicos. https://repositoriodigital.minam.gob.pe/handle/123456789/699
MINAM. (2019b). Mapa Nacional de Ecosistemas del Perú - Memoria Descriptiva. Ministerio Del Ambiente. https://cdn.www.gob.pe/uploads/document/file/309735/Memoria_descriptiva_mapa_Nacional_de_Ecosistemas.pdf
Mind’je, R., Li, L., Nsengiyumva, J. B., Mupenzi, C., Nyesheja, E. M., Kayumba, P. M., Gasirabo, A., & Hakorimana, E. (2020). Landslide susceptibility and influencing factors analysis in Rwanda. Environment, Development and Sustainability, 22(8), 7985–8012. https://doi.org/10.1007/S10668-019-00557-4/METRICS
Molina, A., Vanacker, V., Rosas, M. B., Bonnesoeur, V., Román, F., Ochoa-Tocachi, B., & Buytaert, W. (2019). Infraestructura natural para la gestión de riesgos de erosión e inundaciones en los Andes: ¿Qué sabemos? ForestTrends. https://www.forest-trends.org/wp-content/uploads/2021/06/Infraestructura-natural-para-la-gestion-de-riesgos-de-erosion-e-inundaciones-en-los-Andes.pdf
Monge-Salazar, M. J., Tovar, C., Cuadros-Adriazola, J., Baiker, J. R., Montesinos-Tubée, D. B., Bonnesoeur, V., Antiporta, J., Román-Dañobeytia, F., Fuentealba, B., Ochoa-Tocachi, B. F., & Buytaert, W. (2022). Ecohydrology and ecosystem services of a natural and an artificial bofedal wetland in the central Andes. Science of The Total Environment, 838, 155968. https://doi.org/10.1016/J.SCITOTENV.2022.155968
Mosquera, G. M., Hofstede, R., Bremer, L. L., Asbjornsen, H., Carabajo-Hidalgo, A., et al. (2023). Frontiers in páramo water resources research: A multidisciplinary assessment. Science of The Total Environment, 892, 164373. https://doi.org/https://doi.org/10.1016/j.scitotenv.2023.164373
Naidu, S., Sajinkumar, K. S., Oommen, T., Anuja, V. J., Samuel, R. A., & Muraleedharan, C. (2018). Early warning system for shallow landslides using rainfall threshold and slope stability analysis. Geoscience Frontiers, 9(6), 1871–1882. https://doi.org/10.1016/J.GSF.2017.10.008
Nguyen, T. S., Likitlersuang, S., Ohtsu, H., & Kitaoka, T. (2017). Influence of the spatial variability of shear strength parameters on rainfall induced landslides: a case study of sandstone slope in Japan. Arabian Journal of Geosciences, 10(16), 1–12. https://doi.org/10.1007/S12517-017-3158-Y/FIGURES/16
Ochoa-Sánchez, A., Crespo, P., & Célleri, R. (2018). Quantification of rainfall interception in the high Andean tussock grasslands. Ecohydrology, 11(3), e1946. https://doi.org/https://doi.org/10.1002/eco.1946
Oliva, M., Robert, ;, Torres, P., Salas, R., Gamarra, O., Leiva, S., Collazos, R., Luis, J., & Quintana, M. (2017). Cuantificación del área de pajonal de las microcuencas de Gocta y Chinata y su potencial como reserva de carbono. Scientia Agropecuaria, 8(3), 233–241. https://doi.org/10.17268/sci.agropecu.2017.03.06
Palladino, M. R., Viero, A., Turconi, L., Brunetti, M. T., Peruccacci, S., Melillo, M., Luino, F., Deganutti, A. M., & Guzzetti, F. (2018). Rainfall thresholds for the activation of shallow landslides in the Italian Alps: the role of environmental conditioning factors. Geomorphology, 303, 53–67. https://doi.org/10.1016/J.GEOMORPH.2017.11.009
Pánek, T. (2019). Landslides and Quaternary climate changes—The state of the art. Earth-Science Reviews, 196, 102871. https://doi.org/10.1016/J.EARSCIREV.2019.05.015
Patton, A. I., Rathburn, S. L., & Capps, D. M. (2019). Landslide response to climate change in permafrost regions. Geomorphology, 340, 116–128. https://doi.org/10.1016/J.GEOMORPH.2019.04.029
Peres, D. J., & Cancelliere, A. (2018). Modeling impacts of climate change on return period of landslide triggering. Journal of Hydrology, 567, 420–434. https://doi.org/10.1016/J.JHYDROL.2018.10.036
Perry, L. B., Seimon, A., Andrade-Flores, M. F., Endries, J. L., Yuter, S. E., et al. (2017). Characteristics of Precipitating Storms in Glacierized Tropical Andean Cordilleras of Peru and Bolivia. Annals of the American Association of Geographers, 107(2), 309–322. https://doi.org/10.1080/24694452.2016.1260439
Persichillo, M. G., Bordoni, M., & Meisina, C. (2017). The role of land use changes in the distribution of shallow landslides. Science of The Total Environment, 574, 924–937. https://doi.org/10.1016/J.SCITOTENV.2016.09.125
Pham, B. T., Prakash, I., & Tien Bui, D. (2018). Spatial prediction of landslides using a hybrid machine learning approach based on Random Subspace and Classification and Regression Trees. Geomorphology, 303, 256–270. https://doi.org/10.1016/J.GEOMORPH.2017.12.008
Pham, B. T., Shirzadi, A., Shahabi, H., Omidvar, E., Singh, S. K., et al. (2019). Landslide Susceptibility Assessment by Novel Hybrid Machine Learning Algorithms. Sustainability 2019, Vol. 11, Page 4386, 11(16), 4386. https://doi.org/10.3390/SU11164386
Pham, B. T., Vu, V. D., Costache, R., Phong, T. Van, Ngo, T. Q., Tran, T.-H., Nguyen, H. D., Amiri, M., Tan, M. T., Trinh, P. T., Le, H. Van, & Prakash, I. (2022). Landslide susceptibility mapping using state-of-the-art machine learning ensembles. Geocarto International, 37(18), 5175–5200. https://doi.org/10.1080/10106049.2021.1914746
Pinos-Morocho, D., Morales-Matute, O., & Durán-López, M. E. (2021). Suelos de páramo: Análisis de percepciones de los servicios ecosistémicos y valoración económica del contenido de carbono en la sierra sureste del Ecuador. Revista de Ciencias Ambientales, 55(2), 157–179. https://doi.org/10.15359/RCA.55-2.8
Posner, A. J., & Georgakakos, K. P. (2015). Soil moisture and precipitation thresholds for real-time landslide prediction in El Salvador. Landslides 2015 12:6, 12(6), 1179–1196. https://doi.org/10.1007/S10346-015-0618-X
Reichenbach, P., Busca, C., Mondini, A. C., & Rossi, M. (2014). The Influence of Land Use Change on Landslide Susceptibility Zonation: The Briga Catchment Test Site (Messina, Italy). Environmental Management, 54(6), 1372–1384. https://doi.org/10.1007/S00267-014-0357-0/TABLES/3
Ríos-Touma, B., Rosero, P., Morabowen, A., Guayasamin, J. M., Carson, C., Villamarín-Cortez, S., Solano-Ugalde, A., Tobes, I., & Cuesta, F. (2023). Biodiversity responses to land-use change in the equatorial Andes. Ecological Indicators, 156, 111100. https://doi.org/https://doi.org/10.1016/j.ecolind.2023.111100
Saha, S., Majumdar, P., & Bera, B. (2023). Deep learning and benchmark machine learning based landslide susceptibility investigation, Garhwal Himalaya (India). Quaternary Science Advances, 10, 100075. https://doi.org/https://doi.org/10.1016/j.qsa.2023.100075
Scowen, M., Athanasiadis, I. N., Bullock, J. M., Eigenbrod, F., & Willcock, S. (2021). The current and future uses of machine learning in ecosystem service research. Science of The Total Environment, 799, 149263. https://doi.org/10.1016/J.SCITOTENV.2021.149263
Sobrevilla, L. (2019). Influence of land cover on soil mass movements in the mountains of Apurímac, Peru - CIFOR Knowledge. In AgroParisTech.
Strauch, R., Istanbulluoglu, E., Siddhartha Nudurupati, S., Bandaragoda, C., Gasparini, N. M., & Tucker, G. E. (2018). A hydroclimatological approach to predicting regional landslide probability using Landlab. Earth Surface Dynamics, 6(1), 49–75. https://doi.org/10.5194/ESURF-6-49-2018
Sun, D., Chen, D., Zhang, J., Mi, C., Gu, Q., & Wen, H. (2023). Landslide Susceptibility Mapping Based on Interpretable Machine Learning from the Perspective of Geomorphological Differentiation. Land, 12(5). https://doi.org/10.3390/land12051018
Sun, D., Gu, Q., Wen, H., Xu, J., Zhang, Y., Shi, S., Xue, M., & Zhou, X. (2023). Assessment of landslide susceptibility along mountain highways based on different machine learning algorithms and mapping units by hybrid factors screening and sample optimization. Gondwana Research, 123, 89–106. https://doi.org/https://doi.org/10.1016/j.gr.2022.07.013
Tang, Y., Feng, F., Guo, Z., Feng, W., Li, Z., Wang, J., Sun, Q., Ma, H., & Li, Y. (2020). Integrating principal component analysis with statistically-based models for analysis of causal factors and landslide susceptibility mapping: A comparative study from the loess plateau area in Shanxi (China). Journal of Cleaner Production, 277, 124159. https://doi.org/10.1016/J.JCLEPRO.2020.124159
Tasser, E., Mader, M., & Tappeiner, U. (2003). Effects of land use in alpine grasslands on the probability of landslides. Basic and Applied Ecology, 4(3), 271–280. https://doi.org/10.1078/1439-1791-00153
Tien Bui, D., Hoang, N. D., Nguyen, H., & Tran, X. L. (2019). Spatial prediction of shallow landslide using Bat algorithm optimized machine learning approach: A case study in Lang Son Province, Vietnam. Advanced Engineering Informatics, 42, 100978. https://doi.org/10.1016/J.AEI.2019.100978
Van Beek, L. P. H., & Van Asch, T. W. J. (2004). Regional assessment of the effects of land-use change on landslide hazard by means of physically based modelling. Natural Hazards, 31(1), 289–304. https://doi.org/10.1023/B:NHAZ.0000020267.39691.39/METRICS
Vega-Chuquirimay, E., & Torres-Zuñiga, D. (2013). Manejo Y Conservación De Pasturas Naturales Y Cultivos Temporales. DESCO. https://www.descosur.org.pe/wp-content/uploads/2014/12/Manual008.pdf
Wang, S., Zhang, K., van Beek, L. P. H., Tian, X., & Bogaard, T. A. (2020). Physically-based landslide prediction over a large region: Scaling low-resolution hydrological model results for high-resolution slope stability assessment. Environmental Modelling & Software, 124, 104607. https://doi.org/10.1016/J.ENVSOFT.2019.104607
Wilcox, B. P., Allen, B. L., & Bryant, F. C. (1988). Description and classification of soils of the high-elevation grasslands of central Peru. Geoderma, 42(1), 79–94. https://doi.org/https://doi.org/10.1016/0016-7061(88)90024-9
Wood, J. L., Harrison, S., Reinhardt, L., & Taylor, F. E. (2020). Landslide databases for climate change detection and attribution. Geomorphology, 355, 107061. https://doi.org/10.1016/J.GEOMORPH.2020.107061
Xu, C., Sun, Q., & Yang, X. (2018). A study of the factors influencing the occurrence of landslides in the Wushan area. Environmental Earth Sciences, 77(11), 1–8. https://doi.org/10.1007/S12665-018-7584-2/METRICS
Yu, X., Zhang, K., Song, Y., Jiang, W., & Zhou, J. (2021). Study on landslide susceptibility mapping based on rock–soil characteristic factors. Scientific Reports 2021 11:1, 11(1), 1–27. https://doi.org/10.1038/s41598-021-94936-5
Zari, L., Andrea, C., & Leyda, R. (2019). Pajonales | SPDA Actualidad Ambiental. Sociedad Peruana de Derecho Ambiental. https://www.actualidadambiental.pe/pajonales/
Zhang, T., Fu, Q., Wang, H., Liu, F., Wang, H., & Han, L. (2022). Bagging-based machine learning algorithms for landslide susceptibility modeling. Natural Hazards, 110(2), 823–846. https://doi.org/10.1007/s11069-021-04986-1
Zhang, T., Li, Y., Wang, T., Wang, H., Chen, T., Sun, Z., Luo, D., Li, C., & Han, L. (2022). Evaluation of different machine learning models and novel deep learning-based algorithm for landslide susceptibility mapping. Geoscience Letters, 9(1), 26. https://doi.org/10.1186/s40562-022-00236-9
Zhou, C., Yin, K., Cao, Y., Intrieri, E., Ahmed, B., & Catani, F. (2018). Displacement prediction of step-like landslide by applying a novel kernel extreme learning machine method. Landslides, 15(11), 2211–2225. https://doi.org/10.1007/S10346-018-1022-0/METRICS
Zhu, L., Wang, G., Huang, F., Li, Y., Chen, W., & Hong, H. (2022). Landslide Susceptibility Prediction Using Sparse Feature Extraction and Machine Learning Models Based on GIS and Remote Sensing. IEEE Geoscience and Remote Sensing Letters, 19. https://doi.org/10.1109/LGRS.2021.3054029
Zhuo, L., Dai, Q., Han, D., Chen, N., Zhao, B., & Berti, M. (2019). Evaluation of Remotely Sensed Soil Moisture for Landslide Hazard Assessment. IEEE Journal of Selected Topics in Applied Earth Observations and Remote Sensing, 12(1), 162–173. https://doi.org/10.1109/JSTARS.2018.2883361
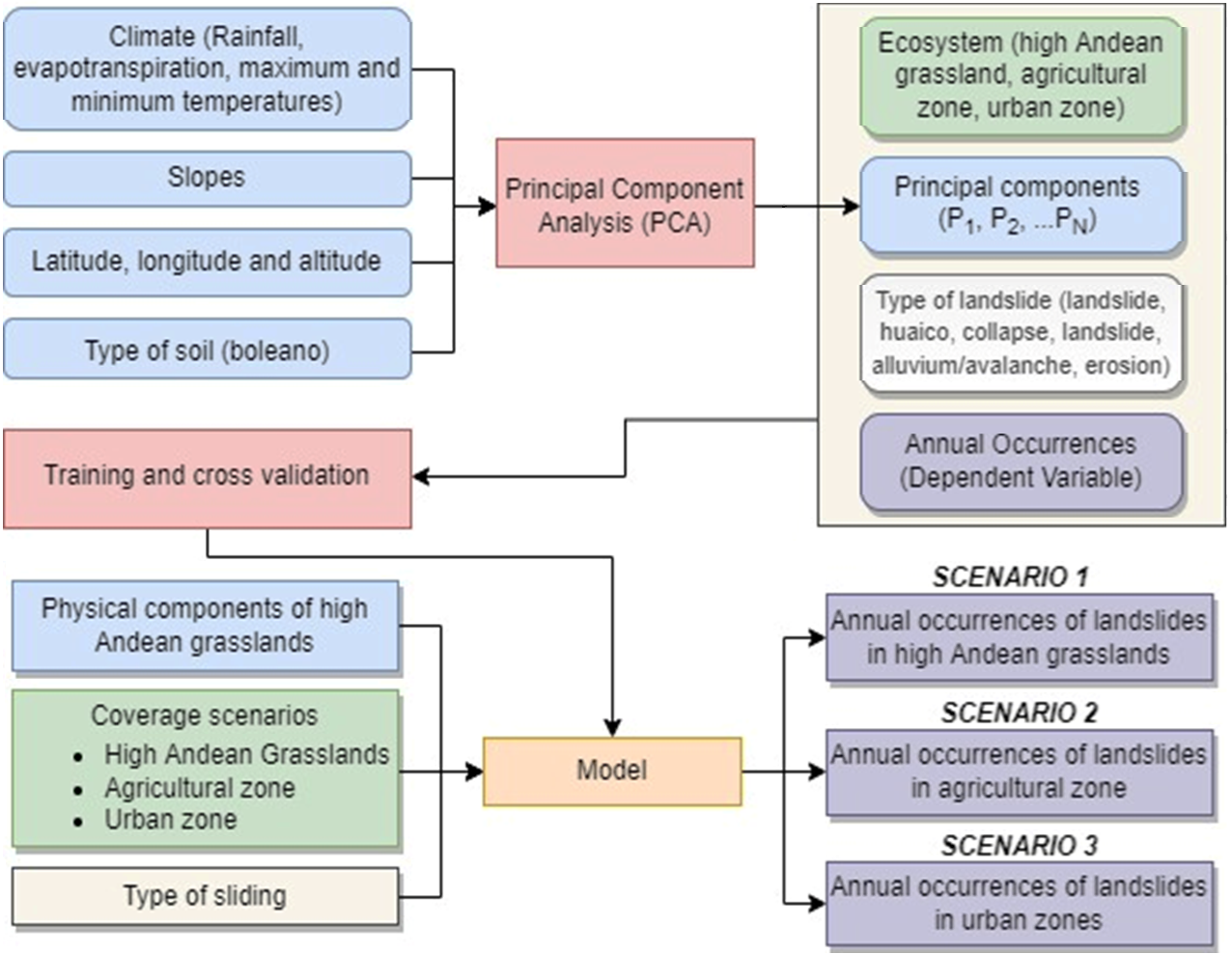
Downloads
Publicado
Como Citar
Edição
Seção
Licença
Copyright (c) 2024 Scientia Agropecuaria

Este trabalho está licenciado sob uma licença Creative Commons Attribution-NonCommercial 4.0 International License.
Los autores que publican en esta revista aceptan los siguientes términos:
a. Los autores conservan los derechos de autor y conceden a la revista el derecho publicación, simultáneamente licenciada bajo una licencia de Creative Commons que permite a otros compartir el trabajo, pero citando la publicación inicial en esta revista.
b. Los autores pueden celebrar acuerdos contractuales adicionales separados para la distribución no exclusiva de la versión publicada de la obra de la revista (por ejemplo, publicarla en un repositorio institucional o publicarla en un libro), pero citando la publicación inicial en esta revista.
c. Se permite y anima a los autores a publicar su trabajo en línea (por ejemplo, en repositorios institucionales o en su sitio web) antes y durante el proceso de presentación, ya que puede conducir a intercambios productivos, así como una mayor citación del trabajo publicado (ver efecto del acceso abierto).