Watershed scale soil moisture estimation model using machine learning and remote sensing in a data-scarce context
DOI:
https://doi.org/10.17268/sci.agropecu.2024.008Palavras-chave:
soil moisture, remote sensing, machine learning, random forest, downscalingResumo
Soil moisture content can be used to predict drought impact on agricultural yield better than precipitation. Remote sensing is viable source of soil moisture data in instrument-scarce areas. However, space-based soil moisture estimates lack suitability for daily and high-resolution agricultural, hydrological, and environmental applications. This study aimed to assess the potential of the random forest machine learning technique to enhance the spatial resolution of remote soil moisture products from the SMAP satellite. Models were built using random forest for spatial downscaling of SMAP-L3-E, then visually and statistically evaluated for disaggregation quality. The impact of topography, soil properties, and precipitation on the downscaled soil moisture was examined. The relationship between downscaled soil moisture and in-situ soil moisture was analyzed. The results indicate that the proposed method demonstrated spatial and hydrological coherence, along with a satisfactory downscaling quality. Statistical validation indicated suitable generalization error for scientific and practical use (RMSE < 0.05 cm3 cm-3). Random forest effectively achieved spatial downscaling of SMAP-L3-E in the study area. Principal component and spatial analysis revealed dependence of downscaled soil moisture on elevation, soil organic carbon content, clay content, and saturated hydraulic conductivity, mainly under near-saturation conditions. Regarding validation against in-situ data, downscaled soil moisture explained in-situ soil moisture well under low soil water content ( = 0.624). Downscaling performance deteriorates for water contents between 0.40 to 0.50 cm3 cm-3, suggesting inadequacy under near saturation conditions at a daily temporal frequency. However, coarser temporal aggregations (7 to 10 days) yielded an average 0.98 correlation coefficient, regardless of saturation conditions. These results could potentially be applied in irrigation planning, soil physics studies and hydrology monitoring, to forecasting the occurrence of droughts, leaching of contaminants, surface runoff modeling, carbon cycle studies, soil's capacity to store and provide nutrients.
Referências
Abbaszadeh, P., Moradkhani, H., & Zhan, X. (2019). Downscaling SMAP Radiometer Soil Moisture Over the CONUS Using an Ensemble Learning Method. Water Resources Research, 55(1), Article 1. https://doi.org/10.1029/2018WR023354
Adab, H., Morbidelli, R., Saltalippi, C., Moradian, M., & Ghalhari, G. A. F. (2020). Machine Learning to Estimate Surface Soil Moisture from Remote Sensing Data. Water, 12(11), Article 11. https://doi.org/10.3390/w12113223
Babaeian, E., Sadeghi, M., Jones, S. B., Montzka, C., Vereecken, H., & Tuller, M. (2019). Ground, Proximal, and Satellite Remote Sensing of Soil Moisture. Reviews of Geophysics, 57(2), Article 2. https://doi.org/10.1029/2018RG000618
Bai, J., Cui, Q., Zhang, W., & Meng, L. (2019). An Approach for Downscaling SMAP Soil Moisture by Combining Sentinel-1 SAR and MODIS Data. Remote Sensing, 11(23), Article 23. https://doi.org/10.3390/rs11232736
Beck, H. E., Pan, M., Miralles, D. G., Reichle, R. H., Dorigo, W. A., Hahn, S., Sheffield, J., Karthikeyan, L., Balsamo, G., Parinussa, R. M., van Dijk, A. I. J. M., Du, J., Kimball, J. S., Vergopolan, N., & Wood, E. F. (2021). Evaluation of 18 satellite- and model-based soil moisture products using in situ measurements from 826 sensors. Hydrology and Earth System Sciences, 25(1), 17-40. https://doi.org/10.5194/hess-25-17-2021
Beven, K., & Freer, J. (2001). A dynamic TOPMODEL. Hydrological Processes, 15(10), 1993-2011. https://doi.org/10.1002/hyp.252
Bivand, R., Keitt, T., & Rowlingson, B. (2021). rgdal: Bindings for the «Geospatial» Data Abstraction Library 1.5-23. http://rgdal.r-forge.r-project.org, https://gdal.org
Breiman, L. (2001). Random Forests. Machine Learning, 45(1), 5-32. https://doi.org/10.1023/A:1010933404324
Brocca, L., Ciabatta, L., Massari, C., Camici, S., & Tarpanelli, A. (2017). Soil Moisture for Hydrological Applications: Open Questions and New Opportunities. Water, 9(2), Article 2. https://doi.org/10.3390/w9020140
Chan, S. K., Bindlish, R., O’Neill, P., Jackson, T., Njoku, E., et al. (2018). Development and assessment of the SMAP enhanced passive soil moisture product. Remote Sensing of Environment, 204, 931-941. https://doi.org/10.1016/j.rse.2017.08.025
Chaubell, J. (2016). Algorithm Theoretical Basis Document SMAP L1B Enhancement Radiometer Brightness Temperature Data Product. 24.
Chen, S., She, D., Zhang, L., Guo, M., & Liu, X. (2019). Spatial Downscaling Methods of Soil Moisture Based on Multisource Remote Sensing Data and Its Application. Water, 11(7), Article 7. https://doi.org/10.3390/w11071401
Chue Hong, N. (2019). How to cite software: Current best practice. https://doi.org/10.5281/ZENODO.2842910
Colliander, A., Jackson, T. J., Bindlish, R., Chan, S., Das, N., Kim, S. B., et al. (2017). Validation of SMAP surface soil moisture products with core validation sites. Remote Sensing of Environment, 191, 215-231. https://doi.org/10.1016/j.rse.2017.01.021
Cooper, D. (2016). Soil water measurement: A practical handbook. John Wiley & Sons.
Crow, W. T., Berg, A. A., Cosh, M. H., Loew, A., Mohanty, B. P., Panciera, R., de Rosnay, P., Ryu, D., & Walker, J. P. (2012). Upscaling sparse ground-based soil moisture observations for the validation of coarse-resolution satellite soil moisture products: UPSCALING SOIL MOISTURE. Reviews of Geophysics, 50(2), Article 2. https://doi.org/10.1029/2011RG000372
Cui, H., Jiang, L., Wang, J., Wang, G., Yang, J., & Su, X. (2019). Downscaling Of SMAP Soil Moisture Products over GENHE Area in China. IGARSS 2019 - 2019 IEEE International Geoscience and Remote Sensing Symposium, 7037-7040. https://doi.org/10.1109/IGARSS.2019.8900144
Das, N. N., Entekhabi, D., Dunbar, R. S., Chaubell, M. J., Colliander, A., Yueh, S., Jagdhuber, T., Chen, F., Crow, W., O’Neill, P. E., Walker, J. P., Berg, A., Bosch, D. D., Caldwell, T., Cosh, M. H., Collins, C. H., Lopez-Baeza, E., & Thibeault, M. (2019). The SMAP and Copernicus Sentinel 1A/B microwave active-passive high resolution surface soil moisture product. Remote Sensing of Environment, 233, 111380. https://doi.org/10.1016/j.rse.2019.111380
Dorigo, W., & de Jeu, R. (2016). Satellite soil moisture for advancing our understanding of earth system processes and climate change. International Journal of Applied Earth Observation and Geoinformation, 48, 1-4. https://doi.org/10.1016/j.jag.2016.02.007
Entekhabi, D., Njoku, E. G., O’Neill, P. E., Kellogg, K. H., Crow, W. T., et al. (2010). The Soil Moisture Active Passive (SMAP) Mission. Proceedings of the IEEE, 98(5), Article 5. https://doi.org/10.1109/JPROC.2010.2043918
Famiglietti, J. S., Ryu, D., Berg, A. A., Rodell, M., & Jackson, T. J. (2008). Field observations of soil moisture variability across scales: SOIL MOISTURE VARIABILITY ACROSS SCALES. Water Resources Research, 44(1), Article 1. https://doi.org/10.1029/2006WR005804
Fang, K., Pan, M., & Shen, C. (2019). The Value of SMAP for Long-Term Soil Moisture Estimation With the Help of Deep Learning. IEEE Transactions on Geoscience and Remote Sensing, 57(4), Article 4. https://doi.org/10.1109/TGRS.2018.2872131
Funk, C., Peterson, P., Landsfeld, M., Pedreros, D., Verdin, J., Shukla, S., Husak, G., Rowland, J., Harrison, L., Hoell, A., & Michaelsen, J. (2015). The climate hazards infrared precipitation with stations—A new environmental record for monitoring extremes. Scientific Data, 2(1), 150066. https://doi.org/10.1038/sdata.2015.66
Gee, G. W., & Or, D. (2002). 2.4 Particle-Size Analysis. En Methods of Soil Analysis: Part IV. Physical Methods (Soil Science Society of America, p. 39).
Grossman, R. B., & Reinsch, T. G. (2002). 2.1 Bulk Density and Linear Extensibility. En Methods of Soil Analysis: Part IV. Physical Methods (Soil Science Society of America, p. 28).
Gruber, S., & Peckham, S. (2009). Land-Surface Parameters and Objects in Hydrology. En Geomorphometry: Concepts, software, applications (1st ed). Elsevier.
Guevara, M., & Vargas, R. (2019). Downscaling satellite soil moisture using geomorphometry and machine learning. PLOS ONE, 14(9), e0219639. https://doi.org/10.1371/journal.pone.0219639
Gupta, S., Papritz, A., Lehmann, P., Hengl, T., Bonetti, S., & Or, D. (2022). Global Soil Hydraulic Properties dataset based on legacy site observations and robust parameterization. Scientific Data, 9(1), 444. https://doi.org/10.1038/s41597-022-01481-5
Gupta, S., Lehmann, P., Bonetti, S., Papritz, A., & Or, D. (2021). Global Prediction of Soil Saturated Hydraulic Conductivity Using Random Forest in a Covariate‐Based GeoTransfer Function (CoGTF) Framework. Journal of Advances in Modeling Earth Systems, 13(4). https://doi.org/10.1029/2020MS002242
Hastie, T., Tibshirani, R., & Friedman, J. (2009). The Elements of Statistical Learning. Springer New York. https://doi.org/10.1007/978-0-387-84858-7
Hengl, T., Mendes de Jesus, J., Heuvelink, G. B. M., Ruiperez Gonzalez, M., et al. (2017). SoilGrids250m: Global gridded soil information based on machine learning. PLOS ONE, 12(2), Article 2. https://doi.org/10.1371/journal.pone.0169748
Hengl, T., Nussbaum, M., Wright, M. N., Heuvelink, G. B. M., & Gräler, B. (2018). Random forest as a generic framework for predictive modeling of spatial and spatio-temporal variables. PeerJ, 6, e5518. https://doi.org/10.7717/peerj.5518
Hernandez-Sanchez, J. C., Monsivais-Huertero, A., Judge, J., & Carlos Jimenez-Escalona, J. (2020). Comparison of SMAP Retrieval Soil Moisture Level 2 Product with in Situ Measurements Over Corn Fields in Central Mexico. IGARSS 2020 - 2020 IEEE International Geoscience and Remote Sensing Symposium, 4727-4730. https://doi.org/10.1109/IGARSS39084.2020.9324106
Heung, B., Ho, H. C., Zhang, J., Knudby, A., Bulmer, C. E., & Schmidt, M. G. (2016). An overview and comparison of machine-learning techniques for classification purposes in digital soil mapping. Geoderma, 265, 62-77. https://doi.org/10.1016/j.geoderma.2015.11.014
Heuvelink, G. B. M., Angelini, M. E., Poggio, L., Bai, Z., Batjes, N. H., Bosch, R., Bossio, D., Estella, S., Lehmann, J., Olmedo, G. F., & Sanderman, J. (2021). Machine learning in space and time for modelling soil organic carbon change. European Journal of Soil Science, 72(4), 1607-1623. https://doi.org/10.1111/ejss.12998
Hu, F., Wei, Z., Zhang, W., Dorjee, D., & Meng, L. (2020). A spatial downscaling method for SMAP soil moisture through visible and shortwave-infrared remote sensing data. Journal of Hydrology, 590, 125360. https://doi.org/10.1016/j.jhydrol.2020.125360
Huang, J., Desai, A. R., Zhu, J., Hartemink, A. E., Stoy, P. C., Loheide, S. P., Bogena, H. R., Zhang, Y., Zhang, Z., & Arriaga, F. (2020). Retrieving Heterogeneous Surface Soil Moisture at 100 m Across the Globe via Fusion of Remote Sensing and Land Surface Parameters. Frontiers in Water, 2, 578367. https://doi.org/10.3389/frwa.2020.578367
Husson, F., Lê, S., & Josse, J. (2008). FactoMineR: An R Package for Multivariate Analysis. Journal of Statistical Software, 25(1), Article 1. https://doi.org/10.18637/jss.v025.i01
Husson, F., Lê, S., & Pagès, J. (2017). Exploratory Multivariate Analysis by Example Using R. CRC Press, 263.
Imfeld, N., Sedlmeier, K., Gubler, S., Correa Marrou, K., Davila, C. P., Huerta, A., Lavado‐Casimiro, W., Rohrer, M., Scherrer, S. C., & Schwierz, C. (2021). A combined view on precipitation and temperature climatology and trends in the southern Andes of Peru. International Journal of Climatology, 41(1), 679-698. https://doi.org/10.1002/joc.6645
Koenker, R., Chernozhukov, V., He, X., & Peng, L. (Eds.). (2017). Handbook of Quantile Regression (1.a ed.). Chapman and Hall/CRC. https://doi.org/10.1201/9781315120256
Krstajic, D., Buturovic, L. J., Leahy, D. E., & Thomas, S. (2014). Cross-validation pitfalls when selecting and assessing regression and classification models. Journal of Cheminformatics, 6(1), 10. https://doi.org/10.1186/1758-2946-6-10
Liu, Y., Jing, W., Wang, Q., & Xia, X. (2020). Generating high-resolution daily soil moisture by using spatial downscaling techniques: A comparison of six machine learning algorithms. Advances in Water Resources, 141, 103601. https://doi.org/10.1016/j.advwatres.2020.103601
Lu, Z., Chai, L., Ye, Q., & Zhang, T. (2015). Reconstruction of time-series soil moisture from AMSR2 and SMOS data by using recurrent nonlinear autoregressive neural networks. 2015 IEEE International Geoscience and Remote Sensing Symposium (IGARSS), 980-983. https://doi.org/10.1109/IGARSS.2015.7325932
Mao, H., Kathuria, D., Duffield, N., & Mohanty, B. P. (2019). Gap Filling of High‐Resolution Soil Moisture for SMAP/Sentinel‐1: A Two‐Layer Machine Learning‐Based Framework. Water Resources Research, 55(8), Article 8. https://doi.org/10.1029/2019WR024902
Mohanty, B. P., Cosh, M. H., Lakshmi, V., & Montzka, C. (2017). Soil Moisture Remote Sensing: State-of-the-Science. Vadose Zone Journal, 16(1), Article 1. https://doi.org/10.2136/vzj2016.10.0105
Montzka, C. M., Cosh, B., Bayat, A., Bitar, A., Berg, R., et al. (2020). Soil Moisture Product Validation Good Practices Protocol. https://doi.org/10.5067/DOC/CEOSWGCV/LPV/SM.001
Nadeem, A. A., Zha, Y., Shi, L., Ali, S., Wang, X., Zafar, Z., Afzal, Z., & Tariq, M. A. U. R. (2023). Spatial Downscaling and Gap-Filling of SMAP Soil Moisture to High Resolution Using MODIS Surface Variables and Machine Learning Approaches over ShanDian River Basin, China. Remote Sensing, 15(3), 812. https://doi.org/10.3390/rs15030812
NASA. (2014). SMAP Handbook Soil Moisture Active Passive. Jet Propulsion Laboratory California Institute of Technology.
Nelson, D. W., & Sommers, L. E. (2018). Total Carbon, Organic Carbon, and Organic Matter. En D. L. Sparks, A. L. Page, P. A. Helmke, R. H. Loeppert, P. N. Soltanpour, M. A. Tabatabai, C. T. Johnston, & M. E. Sumner (Eds.), SSSA Book Series (pp. 961-1010). Soil Science Society of America, American Society of Agronomy. https://doi.org/10.2136/sssabookser5.3.c34
Neteler, M., & Mitasova, H. (2008). Open Source GIS. Springer New York, NY. https://doi.org/10.1007/978-0-387-68574-8
Pebesma, E., & Bivand, R. S. (2005). Classes and Methods for Spatial Data: The sp Package. 21.
Peng, J., Loew, A., Merlin, O., & Verhoest, N. E. C. (2017). A review of spatial downscaling of satellite remotely sensed soil moisture: Downscale Satellite-Based Soil Moisture. Reviews of Geophysics, 55(2), Article 2. https://doi.org/10.1002/2016RG000543
Probst, P., Wright, M. N., & Boulesteix, A. (2019). Hyperparameters and tuning strategies for random forest. WIREs Data Mining and Knowledge Discovery, 9(3). https://doi.org/10.1002/widm.1301
Qin, C., Pei, T., Li, B., Yang, L., & Zhou, C. (2007). An adaptive approach to selecting a flowpartition exponent for a multiple flow direction algorithm. International Journal of Geographical Information Science, 443-458. https://doi.org/10.1080/13658810601073240
Quinn, P. F., Beven, K. J., & Lamb, R. (1995). The in(a/tan/β) index: How to calculate it and how to use it within the topmodel framework. Hydrological Processes, 9(2), Article 2. https://doi.org/10.1002/hyp.3360090204
R Core Team. (2013). R: A language and environment for statistical computing. R Foundation for Statistical Computing [Software]. http://www.R-project.org/
Raduła, M. W., Szymura, T. H., & Szymura, M. (2018). Topographic wetness index explains soil moisture better than bioindication with Ellenberg’s indicator values. Ecological Indicators, 85, 172-179. https://doi.org/10.1016/j.ecolind.2017.10.011
Rao, P., Wang, Y., Wang, F., Liu, Y., Wang, X., & Wang, Z. (2022). Daily soil moisture mapping at 1 km resolution based on SMAP data for areas affected by desertification in Northern China. ESSD, 14(7), 3053–3073. https://doi.org/10.5194/essd-14-3053-2022
Roberts, D. R., Bahn, V., Ciuti, S., Boyce, M. S., Elith, J., et al. (2017). Cross-validation strategies for data with temporal, spatial, hierarchical, or phylogenetic structure. Ecography, 40(8), 913-929. https://doi.org/10.1111/ecog.02881
Sabino Rojas, E., Obando, O. F., & Lavado Casimiro, W. (2017). Atlas de Erosión de Suelos por Regiones Hidrológicas del Perú (Nota Técnica 002). Servicio Nacional de Metereología e Hidrología.
Sagredo, E. A., & Lowell, T. V. (2012). Climatology of Andean glaciers: A framework to understand glacier response to climate change. Global and Planetary Change, 86-87, 101-109. https://doi.org/10.1016/j.gloplacha.2012.02.010
Schratz, P., Becker, M., Lang, M., & Brenning, A. (2021). Mlr3spatiotempcv: Spatiotemporal resampling methods for machine learning in R. arXiv:2110.12674 [Cs, Stat]. http://arxiv.org/abs/2110.12674
SENAMHI. (2015). Validacion de datos CHIRPS de Precipitación para Monitoreo de Periodos Secos y Húmedos en el Perú.
Shangguan, Y., Min, X., Wang, N., Tong, C., & Shi, Z. (2024). A long-term, high-accuracy and seamless 1km soil moisture dataset over the Qinghai-Tibet Plateau during 2001–2020 based on a two-step downscaling method. GIScience & Remote Sensing, 61(1), 2290337. https://doi.org/10.1080/15481603.2023.2290337
Singh, G., Das, N. N., Panda, R. K., Colliander, A., Jackson, T. J., Mohanty, B. P., Entekhabi, D., & Yueh, S. H. (2019). Validation of SMAP Soil Moisture Products Using Ground-Based Observations for the Paddy Dominated Tropical Region of India. IEEE Transactions on Geoscience and Remote Sensing, 57(11), Article 11. https://doi.org/10.1109/TGRS.2019.2921333
Sishah, S., Abrahem, T., Azene, G., Dessalew, A., & Hundera, H. (2023). Downscaling and validating SMAP soil moisture using a machine learning algorithm over the Awash River basin, Ethiopia. PLOS ONE, 18(1), e0279895. https://doi.org/10.1371/journal.pone.0279895
Sumner, M. E., & Miller, W. P. (2018). Cation Exchange Capacity and Exchange Coefficients. En D. L. Sparks, A. L. Page, P. A. Helmke, R. H. Loeppert, P. N. Soltanpour, M. A. Tabatabai, C. T. Johnston, & M. E. Sumner (Eds.), SSSA Book Series (pp. 1201-1229). Soil Science Society of America, American Society of Agronomy. https://doi.org/10.2136/sssabookser5.3.c40
Sun, Q., Miao, C., Duan, Q., Ashouri, H., Sorooshian, S., & Hsu, K. (2018). A Review of Global Precipitation Data Sets: Data Sources, Estimation, and Intercomparisons. Reviews of Geophysics, 56(1), 79-107. https://doi.org/10.1002/2017RG000574
Vergopolan, N., Sheffield, J., Chaney, N. W., Pan, M., Beck, H. E., et al. (2022). High‐Resolution Soil Moisture Data Reveal Complex Multi‐Scale Spatial Variability Across the United States. Geophysical Research Letters, 49(15). https://doi.org/10.1029/2022GL098586
Vergopolan, N., Xiong, S., Estes, L., Wanders, N., Chaney, N. W., et al. (2021). Field-scale soil moisture bridges the spatial-scale gap between drought monitoring and agricultural yields. Hydrology and Earth System Sciences, 25(4), 1827-1847. https://doi.org/10.5194/hess-25-1827-2021
Wackernagel, H. (2010). Multivariate Geostatistics. Springer Berlin Heidelberg. https://doi.org/10.1007/978-3-662-05294-5
Wakigari, S. A., & Leconte, R. (2022). Enhancing Spatial Resolution of SMAP Soil Moisture Products through Spatial Downscaling over a Large Watershed: A Case Study for the Susquehanna River Basin in the Northeastern United States. Remote Sensing, 14(3), 776. https://doi.org/10.3390/rs14030776
Warner, D. L., Guevara, M., Callahan, J., & Vargas, R. (2021). Downscaling satellite soil moisture for landscape applications: A case study in Delaware, USA. Journal of Hydrology: Regional Studies, 38, 100946. https://doi.org/10.1016/j.ejrh.2021.100946
Western, A. W., & Blöschl, G. (1999). On the spatial scaling of soil moisture. Journal of Hydrology, 217(3-4), Article 3-4. https://doi.org/10.1016/S0022-1694(98)00232-7
Wickham, H., François, R., Henry, L., & Müller, K. (2022). dplyr: A Grammar of Data Manipulation. https://dplyr.tidyverse.org
Wright, M. N., & Ziegler, A. (2017). ranger: A Fast Implementation of Random Forests for High Dimensional Data. Journal of Statistical Software, 77(1). https://doi.org/10.18637/jss.v077.i01
Xia, Y., Ek, M. B., Peters-Lidard, C. D., Mocko, D., Svoboda, M., Sheffield, J., & Wood, E. F. (2014). Application of USDM statistics in NLDAS-2: Optimal blended NLDAS drought index over the continental United States: Application of USDM Statistics in NLDAS. Journal of Geophysical Research: Atmospheres, 119(6), 2947-2965. https://doi.org/10.1002/2013JD020994
Xing, C., Chen, N., Zhang, X., & Gong, J. (2017). A Machine Learning Based Reconstruction Method for Satellite Remote Sensing of Soil Moisture Images with In Situ Observations. Remote Sensing, 9(5), Article 5. https://doi.org/10.3390/rs9050484
Xu, C., Ke, J., Zhao, X., & Zhao, X. (2020). Multiscale Quantile Correlation Coefficient: Measuring Tail Dependence of Financial Time Series. Sustainability, 12(12), 4908. https://doi.org/10.3390/su12124908
Xu, J., Su, Q., Li, X., Ma, J., Song, W., Zhang, L., & Su, X. (2024). A Spatial Downscaling Framework for SMAP Soil Moisture Based on Stacking Strategy. Remote Sensing, 16(1), 200. https://doi.org/10.3390/rs16010200
Xu, Y. (2019). Mapping Soil Moisture from Remotely Sensed and In-situ Data with Statistical Methods (p. 100) [Doctoral Dissertation]. Louisiana State University and Agricultural and Mechanical College.
Yamazaki, D., Ikeshima, D., Tawatari, R., Yamaguchi, T., O’Loughlin, F., Neal, J. C., Sampson, C. C., Kanae, S., & Bates, P. D. (2017). A high-accuracy map of global terrain elevations: Accurate Global Terrain Elevation map. Geophysical Research Letters, 44(11), Article 11. https://doi.org/10.1002/2017GL072874
Zappa, L., Forkel, M., Xaver, A., & Dorigo, W. (2019). Deriving Field Scale Soil Moisture from Satellite Observations and Ground Measurements in a Hilly Agricultural Region. Remote Sensing, 11(22), Article 22. https://doi.org/10.3390/rs11222596
Zhao, W., Sánchez, N., Lu, H., & Li, A. (2018). A spatial downscaling approach for the SMAP passive surface soil moisture product using random forest regression. Journal of Hydrology, 563, 1009-1024. https://doi.org/10.1016/j.jhydrol.2018.06.081
Zhu, Z., Bo, Y., & Sun, T. (2023). Spatial downscaling of satellite soil moisture products based on apparent thermal inertia: Considering the effect of vegetation condition. Journal of Hydrology, 616, 128824. https://doi.org/10.1016/j.jhydrol.2022.128824
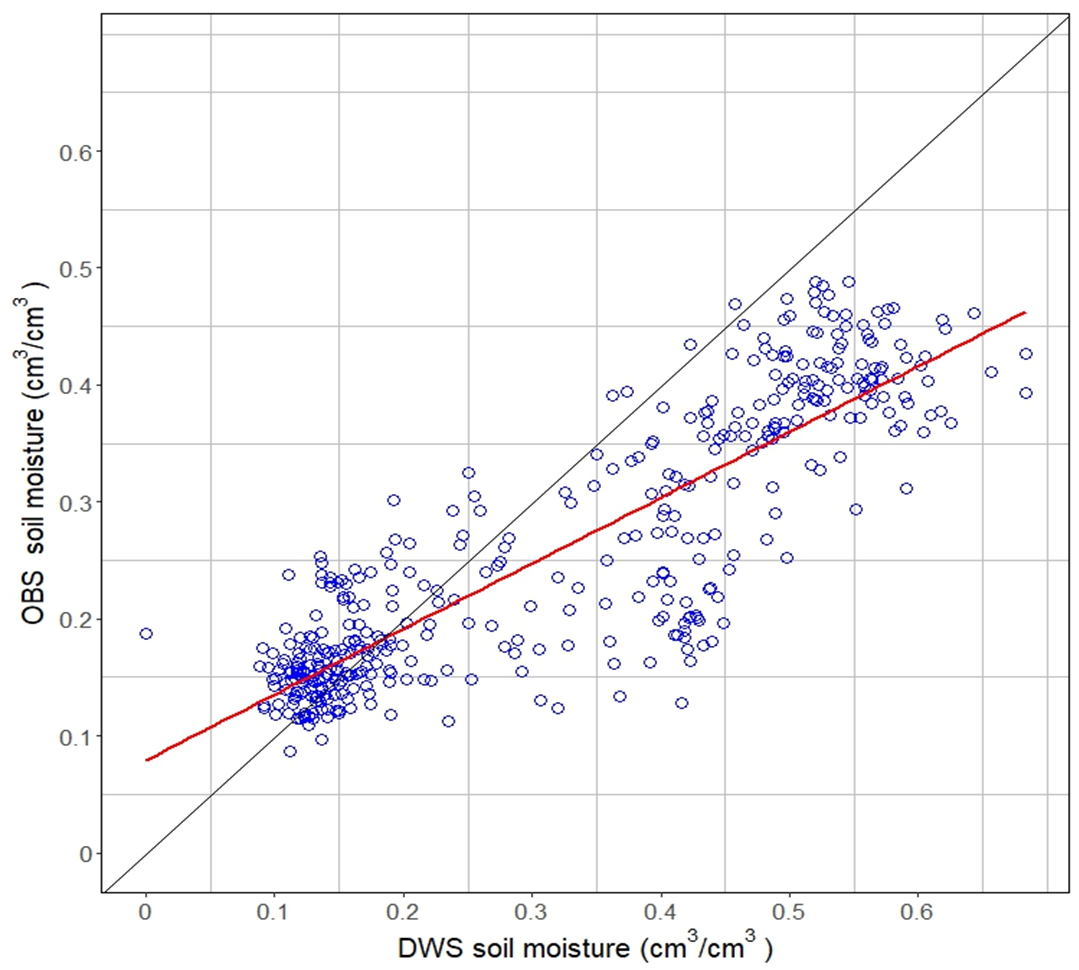
Downloads
Arquivos adicionais
Publicado
Como Citar
Edição
Seção
Licença
Copyright (c) 2024 Scientia Agropecuaria

Este trabalho está licenciado sob uma licença Creative Commons Attribution-NonCommercial 4.0 International License.
Los autores que publican en esta revista aceptan los siguientes términos:
a. Los autores conservan los derechos de autor y conceden a la revista el derecho publicación, simultáneamente licenciada bajo una licencia de Creative Commons que permite a otros compartir el trabajo, pero citando la publicación inicial en esta revista.
b. Los autores pueden celebrar acuerdos contractuales adicionales separados para la distribución no exclusiva de la versión publicada de la obra de la revista (por ejemplo, publicarla en un repositorio institucional o publicarla en un libro), pero citando la publicación inicial en esta revista.
c. Se permite y anima a los autores a publicar su trabajo en línea (por ejemplo, en repositorios institucionales o en su sitio web) antes y durante el proceso de presentación, ya que puede conducir a intercambios productivos, así como una mayor citación del trabajo publicado (ver efecto del acceso abierto).