Estimation of nitrogen content in sugarcane based on vegetation indices derived from Sentinel-2 data
DOI:
https://doi.org/10.17268/sci.agropecu.2025.006Keywords:
agro-model, cultivares, canopy, remote sensing, artificial intelligenceAbstract
Sugarcane occupies a large territorial scale in the world and is constantly searching for mechanisms to monitor nutrients in the crop production cycle, using non-destructive methods. The study aimed to estimate the nitrogen content in the sugarcane leaf was developed in the 2021/2022 harvest on two commercial fields of dryland cultivars (RB867515 = 50.75 ha) and (CVSP7870 = 48.56 ha) at the Serranópolis-Goiás mill, evaluating the efficiency of the biochemical vegetation indices Fraction of Absorbed Photosynthetically Active Radiation (fAPAR) and Canopy Chlorophyll Content (CCC) processed using the radiation transfer model RTM PROSAIL, compared to the Normalized Difference Vegetation Index (NDVI) and Green Normalized Difference Vegetation Index (GNDVI), processed using mathematical band ratio models. Both were based on a time series of Sentinel-2 data as input variables. The validation of the Agro-Model occurred through analysis of leaf tissue collected in seven interspersed evaluations during the period the crop remained in the field. The functionality of the four indexes was evidenced, highlighting the biochemical index fAPAR from the perspective of descriptive statistics (R² = 0.970 and RMSE = 0.46) for the cultivar RB867515 and (R² = 0.940 and RMSE = 0.69) for the cultivar CVSP7870.
References
Ali, A.M., Darvishzadeh, R, Skidmore., A, Gara, T. W., O'Connor, B., Roeoesli, C., Heurich, M., & Paganini, M. (2020). Comparing methods for mapping canopy chlorophyll content in a mixed mountain forest using sentinel-2 data. Int. J. Appl. Earth Obs. Geoinf., 87. https://doi.org/10.1016/j.jag.2019.102037
Andrade, J. B., Ferrari Junior, E., & Possenti, R. A. (2003). Seleção de 39 variedades de cana-de-açúcar para alimentação animal. Brazilian of Journal Veterinary Research Animal Science, 40(4), 287-296. https://doi.org/10.1590/S1413-95962003000400008
Barros, P. P. S., Fiorio, P. R., Demattê, J. A. M., Martins, J. A., Montezano, Z. F., & Dias, F. L. F. (2022). Estimation of leaf nitrogen levels in sugarcane using hyperspectral models. Ciência Rural, Santa Maria, 52(7), e20200630. https://doi.org/10.1590/0103-8478cr20200630
Bassi, M., Menossi, M., & Mattiello, L. (2018). Nitrogen supply influences photosynthesis establishment along the sugarcane leaf. Sci. Rep., 8(1) 2327, https://doi.org/10.1038/s41598-018-20653-1
Berger, K., Verrelst, J., Feret, J.-B., Wang, Z., Wocher, M., Strathmann, M., Danner, M., Mauser, W., & Hank, T. (2020). Crop nitrogen monitoring: Recent progress and principal developments in the contexto of imaging spectroscopy missions. Remote Sensing of Environment, 242, 111758. https://doi.org/10.1016/j.rse.2020.111758
Calheiros, A. S., Oliveira, M. W., Ferreira, V., Barbosa, G. V. S., Costa, J. P. V., Lima, G. S., & Aristides, E. V. S. (2011). Acúmulo de nutrientes e produção de sacarose de duas variedades de cana-de-açúcar na primeira rebrota, em função de doses de fósforo. STAB. Tecnologia/Pesquisa, p. 26-29.
Clevers, J. G. P. W., & Kooistra, L. (2012). Using hyperspectral remote sensing data for retrieving canopy chlorophyll and nitrogen content. IEEE J. Sel. Top. Appl. Earth Observ. 5, 574-583.
Coelho, A.P, Rosalen D. L., & Faria, R. T. (2018). Vegetation indices in the prediction of biomass and grain yield of white oat under irrigation levels. Pesquisa Agropecuária Tropical, 48(2), 109-117. https://doi.org/10.1590/1983-40632018v4851523.
Cremonesi, M. V., Ramalho, B., Golfetto, P., Krepki, L. S., & Pauletti, V. (2019). Marcha de absorção, taxa de acúmulo e exportação de micronutrientes e alumínio pelo tabaco (Nicotiana tabacum L.). Revista de Ciências Agroveterinárias, 18(1), 13-23. https://doi.org/10.5965/223811711812019013.
Darvishzadeh, R., Matkan, A. A., & Dashti Ahangar, A. (2012). Inversion of a radiative transfer model for estimation of rice canopy chlorophyll content using a lookup-table approach. IEEE J. Sel. Top. Appl. Earth Observ. Remote Sens, 5, 1222–1230.
Delloye, C., Weiss, M., & Defourny, P. (2018). Retrieval of the canopy chlorophyll content from sentinel-2 spectral bands to estimate nitrogen uptake in intensive winter wheat cropping systems. Remote Sensing of Environment, 216, 245–261. https://doi.org/10.1016/j.rse.2018.06.037
Gitelson, A. A., Kaufman, Y. J., & Merzlyak, M. N. (1996). Use of a green channel in remote sensing of global vegetation from EOS-MODIS. Remote Sens. Environ., 58, 289–298. https://doi.org/10.1016/S0034-4257(96)00072-7
Gitelson, A. A., & Merzlyak, M. N. (1998). Remote sensing of chlorophyll concentration in higher plant leaves. Adv. Space Res., 22, 689–692.
Houborg, R., Soegaard, H., & Boegh, E. (2007) Combining vegetation index and model inversion methods for the extraction of key vegetation biophysical parameters using Terra and Aqua MODIS reflectance data. Remote Sens Environ, 106, 39–58. https://doi.org/10.1016/j.rse. 2006.07.016
Houlès, V., Mary, B., Machet, J. M., Guérif, M., & Moulin, S. (2001). Do crop characteristics available from remote sensing allow to determine crop nitrogen status? In G. Grenier, & S. Blackmore (Eds.), 3rd European Conferance on Precision Agriculture (pp. 917-922). Montpellier: Agro Montpellier,
Instituto Nacional de Meteorologia - INMET: Banco de dados meteorológicos. https://tempo.inmet.gov.br/
JASP Team (2024). Version 0.18.3) [Computer software]. https://jasp-stats.org/
Liu, W. T. H. (2006). Aplicações de sensoriamento remoto. Campo Grande: Ed. UNIDERP, 908 p.
Martins, J. A., Fiorio, P. R., Silva Barros, P. P., Demattê, J. A. M., Molin, J. P., Cantarella, H., & Neale, C. M. U. (2021). Potential use of hyperspectral data to monitor sugarcane nitrogen status. Acta Scientiarum. Agronomy, 43, e47632. https://doi.org/10.4025/actasciagron.v43i1.47632
Monteith, J. L. (1977). Climate and Efficiency of Crop Production in Britain. Philosophical Transactions of the Royal Society London B, 281, 277-294.
Prado, R. M. (2021). Introduction to Plant Nutrition. In: Mineral nutrition of tropical plants (pp.1-38) Springer, Cham.. https://doi.org/10.1007/978-3-030-71262-4_1
Quaggio, J. A., Cantarella, H., van Raij B, Otto, R., Penatti, C. P., Rossetto, R., et al. (2022). Cana-de-açúcar. In: Boletim 100: recomendações de adubação e calagem para o Estado de São Paulo. Campinas: IAC.
Reyes-Trujillo, A., Daza-Torres, M. C., Galindez-Jamioy, C. A., Rosero-García, E. E., Muñoz-Arboleda, F., & Solarte-Rodriguez, E. (2021). Estimating canopy nitrogen concen-tration of sugarcane crop using in situ spectroscopy, Heliyon, 7(3) e06566, https://doi.org/10.1016/j.heliyon.2021.e06566
Ribeiro, R. V., Machado, R. S., Machado, E. C., Machado, D. F. S. P., Magalhães Filho, J. R., & Landell, M. G. A. (2013). Revealing drought-resistance and productive patterns in sugarcane genotypes by evaluating both physiological responses and stalk yield. Experimental Agriculture, Cambridge, 49, 212-224. https://doi.org/10.1017/S0014479712001263
Rouse, J. W., Haas, R. H., Schell, J. A., & Deering, D. W. (1973). Monitoring the vernal advancement and retrogradation (green wave effect) of natural vegetation. https://ntrs.nasa.gov/citations/19750020419
Stamford, J. D., Vialet-Chabrand, S., Cameron, I., & Lawson, T., (2023). Development of an accurate low cost NDVI imaging system for assessing plant health. Plant Methods, 30, 19. https://doi.org/10.1186/s13007-023-00981-8
Verrelst, J., Malenovsky, Z., Van der Tol, C., Camps-Valls, G., Gastellu-Etchegorry, J. P., Lewis, P., North, P., & Moreno, J. (2019). Quantifying vegetation biophysical variables from imaging spectroscopy data: A review on retrieval methods. Surveys in Geophysics, 40(3), 589–629. https://doi.org/10.1007/s10712-018-9478-y
Vitti, G. C., Luz, P. H. C., & Altran, W. S. (2016). Nutrição e Adubação. p. 66-93. In: Santos, F.; Borém A. Cana-de-Açúcar do Plantio à Colheita. Viçosa: UFV.
Weiss, M., Baret, F., & Sylvain, J. (2020). Sentinel2 ToolBox Level2 Products. V2.0. https://step.esa.int/docs/extra/ATBD_S2ToolBox_V2.0.pdf
Zhao, B., Duan, A., Ata-Ul-Karim, S. T., Liu, Z., Gong, Z., Zhang, J., Xiao, J., liu, Z., Qin, A., & Ning, D. (2018). Exploring new spectral bands and vegetation indices for estimating nitrogen nutrition index of summer maize. European Journal of Agronomy, 93, 113-125. https://doi.org/10.1016/j.eja.2017.12.006
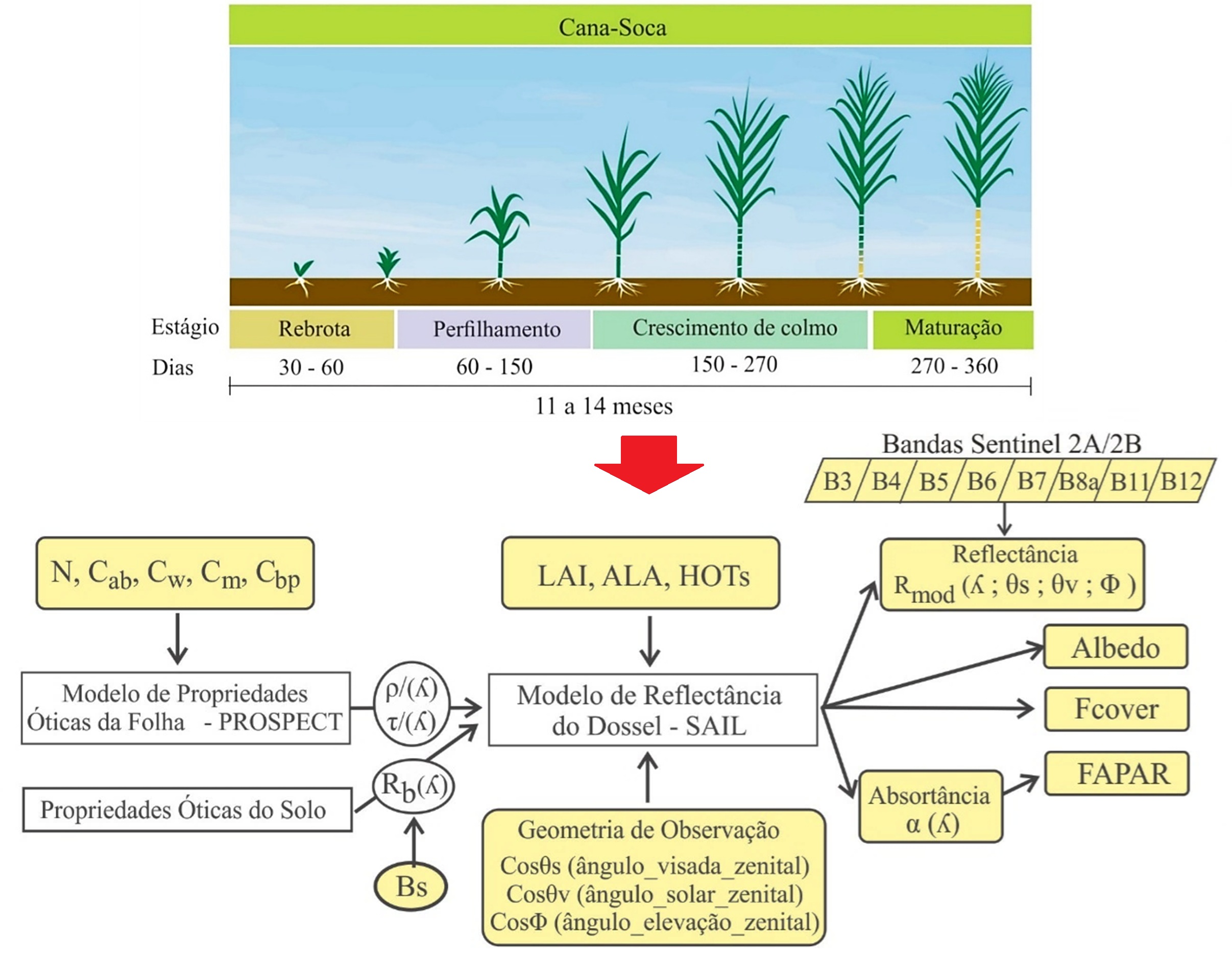
Downloads
Published
How to Cite
Issue
Section
License
Copyright (c) 2025 Scientia Agropecuaria

This work is licensed under a Creative Commons Attribution-NonCommercial 4.0 International License.
The authors who publish in this journal accept the following conditions:
a. The authors retain the copyright and assign to the magazine the right of the first publication, with the work registered with the Creative Commons attribution license, which allows third parties to use the published information whenever they mention the authorship of the work and the First publication in this journal.
b. Authors may make other independent and additional contractual arrangements for non-exclusive distribution of the version of the article published in this journal (eg, include it in an institutional repository or publish it in a book) as long as it clearly indicates that the work Was first published in this journal.
c. Authors are encouraged to publish their work on the Internet (for example, on institutional or personal pages) before and during the review and publication process, as it can lead to productive exchanges and a greater and faster dissemination of work Published (see The Effect of Open Access).