Integración de VANT-LiDAR con imágenes multiespectrales para la estimación del carbono almacenado en plantaciones forestales de Prosopis sp.
DOI:
https://doi.org/10.17268/sci.agropecu.2025.025Palabras clave:
VANT, LiDAR, biomasa, carbono almacenado, índices de vegetaciónResumen
Los individuos del género Prosopis sp. conocidos como algarrobos; son especies claves en el desarrollo del bosque seco y recuperación de áreas degradadas en la Costa norte del Perú. La evaluación de plantaciones, cálculo de la biomasa aérea forestal (BAF) y carbono almacenado representa un papel importante en el manejo forestal y mitigación del cambio climático. Este estudio evalúa metodologías de monitoreo a través del uso de imágenes multiespectrales y LiDAR acopladas a un VANT, con la finalidad de realizar su validación y generar modelos que permitan estimar el carbono almacenado. Se evaluaron siete especies de Prosopis sp. con la metodología convencional y se encontraron diferencias significativas entre las especies para las características dasométricas e índices de vegetación, así como en la comparación con los datos obtenidos con el LiDAR. Se seleccionaron modelos para determinar BAF y la asociación entre el carbono aéreo obtenido con los modelos constituidos por datos de LiDAR e índices de vegetación que presentaron correlaciones significativas (p < 0,05), se construyeron siete modelos para predicción de carbono y destaca el modelo que tiene como variables regresoras la altura total y área de copa obtenidas del LiDAR, así como los índices CIgreen, GNDVI, RECI, LCI y NDVI (R² = 0,77). Lo cual confirma que el uso de la metodología LiDAR con los índices de vegetación permite una estimación más práctica del carbono almacenado en la plantación.
Citas
Al-Jabri, K., Al-Mulla, Y., Al-Abri, A., Al-Battashi, F., Al-Sulaimani, M., Tabook, A., Al-Raba’Ni, S., Sulaiman, H., Al-Salmi, N., & Al-Shukaili, T. (2025). Integrating Remote Sensing Techniques and Allometric Models for Sustainable Carbon Sequestration Estimation in Prosopis cineraria – Druce Trees. Sustainability, 17(1), 123. https://doi.org/10.3390/su17010123
Assefa, D., Mekuriaw, A., Tesfaye, M., Sewnet, H., & Belay, B. (2022). Mapping of Prosopis juliflora rate of expansion and developing species-specific allometric equations to estimate its aboveground biomass in the dry land of Ethiopia. Modeling Earth Systems and Environment, 9(1), 263-274. https://doi.org/10.1007/s40808-022-01495-3
Baloloy, A. B., Blanco, A. C., Candido, C. G., Argamosa, R. J. L., Dumalag, J. B. L. C., Dimapilis, L. L. C., & Paringit, E. C. (2018). Estimation of mangrove forest aboveground biomass using multispectral bands, vegetation indices and biophysical variables derived from optical satellite imageries: Rapideye, Planetscope and Sentinel-2. ISPRS Annals of the Photogrammetry, Remote Sensing and Spatial Information Sciences, IV–3(3), 29–36. https://doi.org/10.5194/isprs-annals-IV-3-29-2018
Beresford-Jones, D. G., T, S. A., Whaley, O. Q., & Chepstow-Lusty, A. J. (2009). The Role ofProsopisin Ecological and Landscape Change in the Samaca Basin, Lower Ica Valley, South Coast Peru from the Early Horizon to the Late Intermediate Period. Latin American Antiquity, 20(2), 303-332. https://doi.org/10.1017/s1045663500002650
Beresford-Jones, D., & Whaley, O. Q. (2021). Prosopis in the history of the coast of Peru. En Elsevier eBooks (pp. 95-103). https://doi.org/10.1016/b978-0-12-823320-7.00012-2
Blackie, R., Baldauf, C., Gautier, D., Gumbo, D., Kassa, H., Parthasarathy, N., Paumgarten, F., Sola, P., Pulla, S., Waeber, P., & Sunderland, T. (2014). Tropical dry forests the state of global knowledge and recommendations for future research.
Brewer, K., Clulow, A., Sibanda, M., Gokool, S., Naiken, V., & Mabhaudhi, T. (2022). Predicting the Chlorophyll Content of Maize over Phenotyping as a Proxy for Crop Health in Smallholder Farming Systems. Remote Sensing, 14(3), 518. https://doi.org/10.3390/rs14030518
Bueno, G. F., Costa, E. A., Finger, C. A. G., Liesenberg, V., & Bispo, P. da C. (2022). Machine Learning: Crown Diameter Predictive Modeling for Open-Grown Trees in the Cerrado Biome, Brazil. Forests, 13(8), 1295. https://doi.org/10.3390/f13081295
Candiago, S., Remondino, F., De Giglio, M., Dubbini, M., & Gattelli, M. (2015). Evaluating Multispectral Images and Vegetation Indices for Precision Farming Applications from UAV Images. Remote Sensing, 7(4), 4026–4047. https://doi.org/10.3390/rs70404026
Cárdenas-Rengifo, G. P., Baselly-Villanueva, J. R., Chumbimune-Vivanco, S. Y., Macedo-Ramírez, A. T., Salazar, E., Minaya, B., Quintana, S., Cabudivo, A., Palma, S. S. A., Álvarez-Álvarez, P., & Ocaña-Reyes, J. A. (2024). Using acoustic tomography to model wood deterioration in Cedrelinga cateniformis Ducke in the Peruvian Amazon. Forests, 15(5), 778. https://doi.org/10.3390/f15050778
Carpp, I. A., Sione, S. M. J., Ledesma, S. G., Oszust, J. D., & Rosenberger, L. J. (2017). Factores de expansión de la biomasa de Prosopis affinis (spreng.), como herramienta para la estimación del carbono almacenado en bosques nativos del espinal (Entre Ríos, Argentina).
Castillo, R. (2022). Actualización del conocimiento de las especies de Prosopis (Fabaceae-Mimosoideae) que crecen en Chile. Revista Chilena de Flora y de Vegetación, 25(1), 11–55.
Chicco, D., Warrens, M. J., & Jurman, G. (2021). The coefficient of determination R-squared is more informative than SMAPE, MAE, MAPE, MSE and RMSE in regression analysis evaluation. PeerJ Computer Science, 7, e623. https://doi.org/10.7717/peerj-cs.623
Chung, C. H., Wang, C. H., Hsieh, H. C., & Huang, C. Y. (2019). Comparison of forest canopy height profiles in a mountainous region of Taiwan derived from airborne lidar and unmanned aerial vehicle imagery. GIScience and Remote Sensing, 56(8), 1289–1304. https://doi.org/10.1080/15481603.2019.1627044
Cisneros, A. B., Moglia, J. G., & Álvarez, J. A. (2019). Morfometria de copa en Prosopis alba; Griseb. Ciência Florestal, 29(2), 863–884. https://doi.org/10.5902/1980509826846
Colomina, I., & Molina, P. (2014). Unmanned aerial systems for photogrammetry and remote sensing: A review. ISPRS Journal of Photogrammetry and Remote Sensing, 92, 79–97. https://doi.org/10.1016/j.isprsjprs.2014.02.013
Corti, M., Cavalli, D., Cabassi, G., Bechini, L., Pricca, N., Paolo, D., Marinoni, L., Vigoni, A., Degano, L., & Marino Gallina, P. (2022). Improved estimation of herbaceous crop aboveground biomass using UAV-derived crop height combined with vegetation indices. Precision Agriculture, 24(2), 587–606. https://doi.org/10.1007/s11119-022-09960-w
Calvo-Rodriguez, S., Sánchez-Azofeifa, G. A., Durán, S. M., Do Espírito-Santo, M. M., & Ferreira Nunes, Y. R. (2021). Dynamics of Carbon Accumulation in Tropical Dry Forests under Climate Change Extremes. Forests, 12(1), 106. https://doi.org/10.3390/f12010106
Dalla Corte, A. P., Rex, F. E., Almeida, D. R. A. de, Sanquetta, C. R., Silva, C. A., Moura, M. M., Wilkinson, B., Zambrano, A. M. A., Cunha Neto, E. M. da, Veras, H. F. P., Moraes, A. de, Klauberg, C., Mohan, M., Cardil, A., & Broadbent, E. N. (2020). Measuring Individual Tree Diameter and Height Using GatorEye High-Density UAV-Lidar in an Integrated Crop-Livestock-Forest System. Remote Sensing, 12(5), 863. https://doi.org/10.3390/rs12050863
Duff, A. B., Meyer, J. M., Pollock, C., & Felker, P. (1994). Biomass production and diameter growth of nine half-sib families of mesquite (Prosopis glandulosa var. glandulosa) and a fast growing Prosopis alba half-sib family grown in Texas. Forest Ecology and Management, 67(1–3), 257–266. https://doi.org/10.1016/0378-1127(94)90020-5
Eitel, J. U. H., Vierling, L. A., Litvak, M. E., Long, D. S., Schulthess, U., Ager, A. A., Krofcheck, D. J., & Stoscheck, L. (2011). Broadband, red-edge information from satellites improves early stress detection in a New Mexico conifer woodland. Remote Sensing of Environment, 115(12), 3640–3646. https://doi.org/10.1016/j.rse.2011.09.002
Fontana, M. L. (2020). Distribución, bioecología y provisión de bienes y servicios ecosistémicos de Prosopis alba en Argentina. Revista de La Facultad de Agronomía, 119(2), 050. https://doi.org/10.24215/16699513e050
Gallardo-Salazar, J. L., Pompa-García, M., Aguirre-Salado, C. A., López-Serrano, P. M., & Meléndez-Soto, A. (2020). Drones: tecnología con futuro promisorio en la gestión forestal. Revista Mexicana de Ciencias Forestales, 11(61). https://doi.org/10.29298/rmcf.v11i61.794
Ganz, S., Käber, Y., & Adler, P. (2019). Measuring tree height with remote sensing-a comparison of photogrammetric and LiDAR data with different field measurements. Forests, 10(8). https://doi.org/10.3390/f10080694
Gitelson, A. A., Viña, A., Ciganda, V., Rundquist, D. C., & Arkebauer, T. J. (2005). Remote estimation of canopy chlorophyll content in crops. Geophysical Research Letters, 32(8), 1–4. https://doi.org/10.1029/2005GL022688
Guariguata, M. R, Arce, J., Ammour, T., & Capella J. L. (2017). Las plantaciones forestales en Perú: Reflexiones, estatus actual y perspectivas a futuro. Center for International Forestry Research (CIFOR). https://doi.org/10.17528/cifor/006461
Islas-Gutiérrez, F., Cruz-Juárez, E., Buendía-Rodríguez, E., Guerra-De la Cruz, V., Pineda-Ojeda, T., Flores-Ayala, E., Carrillo-Anzures, F., & Acosta-Mireles, M. (2024). Ecuación alométrica para estimar biomasa aérea de árboles de Pinus hartwegii lindl. a partir de datos LiDAR. Revista Fitotecnia Mexicana, 47(1), 70. https://doi.org/10.35196/rfm.2024.1.70
Jain, N., Ray, S. S., Singh, J. P., & Panigrahy, S. (2007). Use of hyperspectral data to assess the effects of different nitrogen applications on a potato crop. Precision Agriculture, 8(4–5), 225–239. https://doi.org/10.1007/s11119-007-9042-0
Jarahizadeh, S., & Salehi, B. (2025). Advancing Tree Detection in Forest Environments: A Deep Learning Object Detector Approach with UAV LiDAR Data. Urban Forestry & Urban Greening, 128695. https://doi.org/10.1016/j.ufug.2025.128695
Li, T., Lin, J., Wu, W., & Jiang, R. (2024). Effects of Illumination Conditions on Individual Tree Height Extraction Using UAV LiDAR: Pilot Study of a Planted Coniferous Stand. Forests, 15(5), 758. https://doi.org/10.3390/f15050758
Li, W., Niu, Z., Liang, X., Li, Z., Huang, N., Gao, S., Wang, C., & Muhammad, S. (2015). Geostatistical modeling using LiDAR-derived prior knowledge with SPOT-6 data to estimate temperate forest canopy cover and above-ground biomass via stratified random sampling. International Journal of Applied Earth Observation and Geoinformation, 41, 88–98. https://doi.org/10.1016/j.jag.2015.04.020
Liao, K., Li, Y., Zou, B., Li, D., & Lu, D. (2022). Examining the Role of UAV Lidar Data in Improving Tree Volume Calculation Accuracy. Remote Sensing, 14(17), 4410. https://doi.org/10.3390/rs14174410
Liao, L., Cao, L., Xie, Y., Luo, J., & Wang, G. (2022). Phenotypic Traits Extraction and Genetic Characteristics Assessment of Eucalyptus Trials Based on UAV-Borne LiDAR and RGB Images. Remote Sensing, 14(3). https://doi.org/10.3390/rs14030765
Lu, H., Fan, T., Ghimire, P., & Deng, L. (2020). Experimental Evaluation and Consistency Comparison of UAV Multispectral Minisensors. Remote Sensing, 12(16), 2542. https://doi.org/10.3390/rs12162542
Matese, A., Toscano, P., Di Gennaro, S., Genesio, L., Vaccari, F., Primicerio, J., Belli, C., Zaldei, A., Bianconi, R., & Gioli, B. (2015). Intercomparison of UAV, Aircraft and Satellite Remote Sensing Platforms for Precision Viticulture. Remote Sensing, 7(3), 2971–2990. https://doi.org/10.3390/rs70302971
Méndez, J., Turlan, O., Rios, J., Nájera, J. (2012). Ecuaciones alométricas para estimar biomasa aérea de Prosopis laevigata (Humb. & Bonpl. ex Willd.) M.C. Johnst. Revista Mexicana de Ciencias Forestales, 3.
Meng, J., Xu, J., & You, X. (2015). Optimizing soybean harvest date using HJ-1 satellite imagery. Precision Agriculture, 16(2), 164–179. https://doi.org/10.1007/s11119-014-9368-3
Modica, G., Messina, G., De Luca, G., Fiozzo, V., & Praticò, S. (2020). Monitoring the vegetation vigor in heterogeneous citrus and olive orchards. A multiscale object-based approach to extract trees’ crowns from UAV multispectral imagery. Computers And Electronics In Agriculture, 175, 105500. https://doi.org/10.1016/j.compag.2020.105500
Mohan, M., Silva, C., Klauberg, C., Jat, P., Catts, G., Cardil, A., Hudak, A., & Dia, M. (2017). Individual Tree Detection from Unmanned Aerial Vehicle (UAV) Derived Canopy Height Model in an Open Canopy Mixed Conifer Forest. Forests, 8(9), 340. https://doi.org/10.3390/f8090340
Muturi, G. M., Kariuki, J. C., Poorter, L., & Mohren, G. M. J. (2011). Allometric equations for estimating biomass in naturally established Prosopis stands in Kenya. Journal of Horticulture and Forestry, 4(4). https://doi.org/10.5897/JHF11.066
Padrón, E., & Navarro, R. M. (2004). Estimation of above-ground biomass in naturally occurring populations of Prosopis pallida (H. & B. ex. Willd.) H.B.K. in the north of Peru. Journal of Arid Environments, 56(2), 283–292. https://doi.org/10.1016/S0140-1963(03)00055-7
Paladines, R. (2003). Propuesta de conservación del Bosque seco en el Sur de Ecuador. Lyonia, 4(2), 183–186.
Pearse, G. D., Dash, J. P., Persson, H. J., & Watt, M. S. (2018). Comparison of high-density LiDAR and satellite photogrammetry for forest inventory. ISPRS Journal of Photogrammetry and Remote Sensing, 142, 257–267. https://doi.org/10.1016/j.isprsjprs.2018.06.006
Phadikar, S., & Goswami, J. (2016). Vegetation indices based segmentation for automatic classification of brown spot and blast diseases of rice. 2016 3rd International Conference on Recent Advances in Information Technology (RAIT), 284–289. https://doi.org/10.1109/RAIT.2016.7507917
Plaza, H. (2020). Niveles de vigorosidad de plantaciones de Teca (Tectona grandis), mediante índices de vegetación, en cantón Buena Fe, año 2020 [Master’s thesis]. Universidad Técnica Estatal de Quevedo.
Poley, L., & McDermid, G. (2020). A Systematic Review of the Factors Influencing the Estimation of Vegetation Aboveground Biomass Using Unmanned Aerial Systems. Remote Sensing, 12(7), 1052. https://doi.org/10.3390/rs12071052
Pourazar, H., Samadzadegan, F., & Dadrass Javan, F. (2019). Aerial multispectral imagery for plant disease detection: radiometric calibration necessity assessment. European Journal of Remote Sensing, 52(sup3), 17–31. https://doi.org/10.1080/22797254.2019.1642143
R Core Team. (2020). R: A language and environment for statistical computing. R Foundation for Statistical Computing. https://www.R-project.org/
Raes, D., Fereres, E., García Vila, M., Curnel, Y., Knoden, D., Çelik, S. K., Ucar, Y., Türk, M., & Wellens, J. (2023). Simulation of alfalfa yield with AquaCrop. Agricultural Water Management, 284, 108341. https://doi.org/10.1016/j.agwat.2023.108341
Remache-Reinoso, F. M., Horna-Durán, S. D., Lara-Vásconez, N. X., Cushquicullma-Colcha, D. F., & Muñoz-Jácome, E. A. (2024). Estimación de alturas de pinus radiata d. Don en san juan, chimborazo, usando vehículos aéreos no tripulados. Perfiles, 1(32), 6-14. https://doi.org/10.47187/perf.v1i32.276
Reis-Pereira, M., Verrelst, J., Tosin, R., Rivera Caicedo, J. P., Tavares, F., Neves dos Santos, F., & Cunha, M. (2024). Plant Disease Diagnosis Based on Hyperspectral Sensing: Comparative Analysis of Parametric Spectral Vegetation Indices and Nonparametric Gaussian Process Classification Approaches. Agronomy, 14(3), 493. https://doi.org/10.3390/agronomy14030493
Rodríguez-Puerta, F., Gómez-García, E., Martín-García, S., Pérez-Rodríguez, F., & Prada, E. (2021). UAV-Based LiDAR Scanning for Individual Tree Detection and Height Measurement in Young Forest Permanent Trials. Remote Sensing, 14(1), 170. https://doi.org/10.3390/rs14010170
Salazar, P., Navarro-Cerrillo, R. M., Palacios Mc Cubbin, E., Cruz, G., & Lopez, M. (2023). Biomass estimation models for four priority Prosopis species: Tools required for forestry management in overexploited arid ecosystems. Journal of Arid Environments, 209, 104904. https://doi.org/10.1016/j.jaridenv.2022.104904
Sione, S., Ledesma, S., Rosenberger, L., Oszust, J., Andrade-Castañeda, H., Maciel, G., Wilson, M., & Sasal, M. (2020). Allometric equations of aerial biomass for Prosopis nigra (Griseb.) Hieron." Algarrobo negro"(Fabaceae) in forests of Entre Ríos (Argentina). Rev. Facultad de Agronomía UBA, 40(1), 63–76.
Sione, S. M. J., Andrade-Castañeda, H. J., Ledesma, S. G., Rosenberger, L. J., Oszust, J. D., & Wilson, M. G. (2019). Aerial biomass allometric models for Prosopis affinis Spreng. in native Espinal forests of Argentina. Revista Brasileira de Engenharia Agrícola e Ambiental, 23(6), 467–473. https://doi.org/10.1590/1807-1929/agriambi.v23n6p467-473
Su, R., Du, W., Ying, H., Shan, Y., & Liu, Y. (2023). Estimation of Aboveground Carbon Stocks in Forests Based on LiDAR and Multispectral Images: A Case Study of Duraer Coniferous Forests. Forests, 14(5), 992. https://doi.org/10.3390/f14050992
Tafur, E., Veneros, J., García, L., Gamarra, Ó., Farje, J., & Santistevan, M. (2022). Técnicas no destructivas para la estimación de la biomasa forestal aérea. Idesia (Arica), 40(3), 7–17. https://doi.org/10.4067/S0718-34292022000300007
Taubert, F., Fischer, R., Knapp, N., & Huth, A. (2021). Deriving Tree Size Distributions of Tropical Forests from Lidar. Remote Sensing, 13(1), 131. https://doi.org/10.3390/rs13010131
Vashum, K., & Jayakumar, S. (2012). Methods to Estimate Above-Ground Biomass and Carbon Stock in Natural Forests - A Review. Journal of Ecosystem & Ecography, 2(4). https://doi.org/10.4172/2157-7625.1000116
Vera, E., Cruz, C., Barboza, E., Salazar, W., Canta, J., Salazar, E., Vásquez, H. V. & Arbizu, C. I. (2024). Change of vegetation cover and land use of the Pómac forest historical sanctuary in northern Peru. International Journal of Environmental Science and Technology, 1-12. https://doi.org/10.1007/s13762-024-05597-6
Walker, S. M., Murray, L., & Tepe, T. (2016). Allometric Equation Evaluation Guidance Document. Winrock International, USA.
Wallace, L., Watson, C., & Lucieer, A. (2014). Detecting pruning of individual stems using Airborne Laser Scanning data captured from an Unmanned Aerial Vehicle. International Journal of Applied Earth Observation and Geoinformation, 30(1), 76–85. https://doi.org/10.1016/j.jag.2014.01.010
Xu, W., Cheng, Y., Luo, M., Mai, X., Wang, W., Zhang, W., & Wang, Y. (2025). Progress and Limitations in Forest Carbon Stock Estimation Using Remote Sensing Technologies: A Comprehensive Review. Forests, 16(3), 449. https://doi.org/10.3390/f16030449
Xu, W., Su, Z., Feng, Z., Xu, H., Jiao, Y., & Yan, F. (2013). Comparison of conventional measurement and LiDAR-based measurement for crown structures. Computers and Electronics in Agriculture, 98, 242–251. https://doi.org/10.1016/j.compag.2013.08.015
Zhai, Y., Wang, L., Yao, Y., Jia, J., Li, R., Ren, Z., He, X., Ye, Z., Zhang, X., Chen, Y., & Xu, Y. (2024). Spatially continuous estimation of urban forest aboveground biomass with UAV-LiDAR and multispectral scanning: An allometric model of forest structural diversity. Agricultural And Forest Meteorology, 360, 110301. https://doi.org/10.1016/j.agrformet.2024.110301
Zhang, L., Zhao, Y., Chen, C., Li, X., Mao, F., Lv, L., Yu, J., Song, M., Huang, L., Chen, J., Zheng, Z., & Du, H. (2024). UAV-LiDAR Integration with Sentinel-2 Enhances Precision in AGB Estimation for Bamboo Forests. Remote Sensing, 16(4), 705. https://doi.org/10.3390/rs16040705
Zeng, H.-Q., Liu, Q.-J., Feng, Z.-W., & Ma, Z.-Q. (2010). Biomass equations for four shrub species in subtropical China. Journal of Forest Research, 15(2), 83–90. https://doi.org/10.1007/s10310-009-0150-8
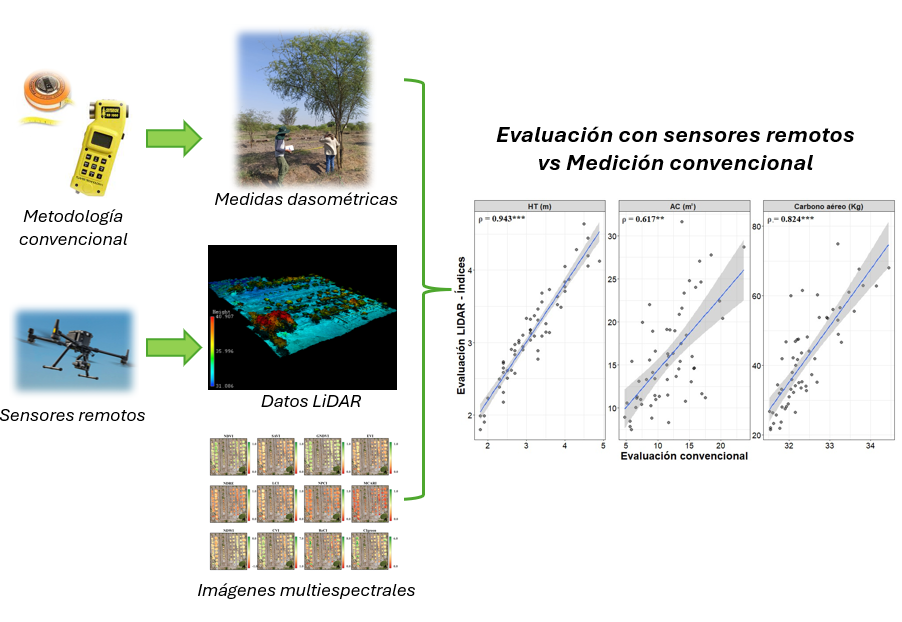
Descargas
Publicado
Cómo citar
Número
Sección
Licencia
Derechos de autor 2025 Scientia Agropecuaria

Esta obra está bajo una licencia internacional Creative Commons Atribución-NoComercial 4.0.
Los autores que publican en esta revista aceptan los siguientes términos:
a. Los autores conservan los derechos de autor y conceden a la revista el derecho publicación, simultáneamente licenciada bajo una licencia de Creative Commons que permite a otros compartir el trabajo, pero citando la publicación inicial en esta revista.
b. Los autores pueden celebrar acuerdos contractuales adicionales separados para la distribución no exclusiva de la versión publicada de la obra de la revista (por ejemplo, publicarla en un repositorio institucional o publicarla en un libro), pero citando la publicación inicial en esta revista.
c. Se permite y anima a los autores a publicar su trabajo en línea (por ejemplo, en repositorios institucionales o en su sitio web) antes y durante el proceso de presentación, ya que puede conducir a intercambios productivos, así como una mayor citación del trabajo publicado (ver efecto del acceso abierto).