Identificación del estado fitosanitario de árboles mediante aprendizaje automático e imágenes de muy alta resolución espacial
DOI:
https://doi.org/10.17268/sci.agropecu.2024.013Palabras clave:
agricultura de precisión, árboles enfermos, cítricos; naranja, México, vehículo aéreo no tripuladoResumen
Las enfermedades de los árboles contribuyen a importantes pérdidas económicas y de alimentos en el sector agrícola. La detección temprana de problemas fitosanitarios en árboles con métodos no destructivos resulta fundamental para garantizar la producción sostenible de naranja. Este trabajo presenta los resultados de una metodología diseñada para la identificación de árboles de naranja enfermos en una huerta ubicada en el cinturón citrícola de México, particularmente en la región de Rioverde, San Luis Potosí. Para ello, se tomaron imágenes con una cámara multiespectral de muy alta resolución espacial instalada en un vehículo aéreo no tripulado con las que se construyó un ortomosaico georreferenciado. Se emplearon seis clases temáticas para identificar los diferentes niveles de sanidad. Se utilizaron diferentes algoritmos de clasificación supervisada a nivel píxel que incluyen Random Forest (RF), K-Nearest Neighbor (KNN), Spectral Angle Mapper (SAM), Support Vector Machine (SVM), y Maximum Likelihood (ML). Considerando la exactitud de clasificación obtenida por cada uno de los algoritmos, se pueden ordenar de la siguiente manera: Maximum Likelihood (ML) con un 88,10%, Support Vector Machine (SVM) con un 77,38%, Spectral Angle Mapper (SAM) con un 76,19%, K-Nearest Neighbor (KNN) con un 64,68% y Random Forest (RF) con un 61,90%. Los resultados permitieron identificar el estado fitosanitario de todos los árboles de la huerta, con una exactitud aceptable y representan información valiosa de manejo para el productor.
Citas
Abdulridha, J., Batuman, O., & Ampatzidis, Y. (2019). UAV-Based Remote Sensing Technique to Detect Citrus Canker Disease Utilizing Hyperspectral Imaging and Machine Learning. Remote Sensing, 11(11), 1373. https://doi.org/10.3390/rs11111373
Ali, H., Lali, M. I., Nawaz, M. Z., Sharif, M., & Saleem, B. A. (2017). Symptom based automated detection of citrus diseases using color histogram and textural descriptors. Computers and Electronics in Agriculture, 138, 92-104. https://doi.org/10.1016/j.compag.2017.04.008
Alzubi, E., Shbikat, N., & Noche, B. (2023). A system dynamics model to improving sustainable performance of the citrus farmers in Jordan Valley. Cleaner Production Letters, 4, 100034. https://doi.org/10.1016/j.clpl.2023.100034
Ampatzidis, Y., Partel, V., & Costa, L. (2020). Agroview: Cloud-based application to process, analyze and visualize UAV-collected data for precision agriculture applications utilizing artificial intelligence. Computers and Electronics in Agriculture, 174, 105457. https://doi.org/10.1016/j.compag.2020.105457
Antolínez, C.A., Olarte-Castillo, X.A., Martini, X., & Rivera, M.J. (2022). Influence of daily temperature maximums on the development and short-distance movement of the Asian citrus psyllid. Journal of Thermal Biology, 110, 103354. https://doi.org/10.1016/j.jtherbio.2022.103354
Atta, S., Zhou, C., Zhou, Y., Cao, M., & Wang, X. (2012). Distribution and Research Advances of Citrus tristeza virus. Journal of Integrative Agriculture, 11(3), 346-358. https://doi.org/10.1016/S2095-3119(12)60019-7
Balfagón, D., Arbona, V., & Gómez-Cadenas, A. (2021). El futuro de los cítricos: Impacto del cambio climático en la citricultura. Metode Science Studies Journal, 110, 61-67. https://doi.org/10.7203/metode.12.20319
Barman, U., & Choudhury, R. D. (2022). Smartphone assist deep neural network to detect the citrus diseases in Agri-informatics. Global Transitions Proceedings, 3(2), 392-398. https://doi.org/10.1016/j.gltp.2021.10.004
Bassanezi, R. B., Primiano, I. V., & Vescove, H. V. (2021). Effect of enhanced nutritional programs and exogenous auxin spraying on huanglongbing severity, fruit drop, yield and economic profitability of orange orchards. Crop Protection, 145, 105609. https://doi.org/10.1016/j.cropro.2021.105609
Berk, Z. (2016). Chapter 5 - Diseases and pests. Citrus Fruit Processing. 83-93. https://doi.org/10.1016/B978-0-12-803133-9.00005-9
Braham, M., Boulahia-Kheder, S., Kahia, M., & Nouira, S. (2023). Aphids and citrus responses to nitrogen fertilization. Journal of the Saudi Society of Agricultural Sciences, S1658077X23000322. https://doi.org/10.1016/j.jssas.2023.03.003
Calou, V. B. C., Teixeira, A. D. S., Moreira, L. C. J., Lima, C. S., De Oliveira, J. B., & De Oliveira, M. R. R. (2020). The use of UAVs in monitoring yellow sigatoka in banana. Biosystems Engineering, 193, 115-125. https://doi.org/10.1016/j.biosystemseng.2020.02.016
Chang, A., Yeom, J., Jung, J., & Landivar, J. (2020). Comparison of Canopy Shape and Vegetation Indices of Citrus Trees Derived from UAV Multispectral Images for Characterization of Citrus Greening Disease. Remote Sensing, 12(24), 4122. https://doi.org/10.3390/rs12244122
Deng, X., Huang, Z., Zheng, Z., Lan, Y., & Dai, F. (2019). Field detection and classification of citrus Huanglongbing based on hyperspectral reflectance. Computers and Electronics in Agriculture, 167, 105006. https://doi.org/10.1016/j.compag.2019.105006
El Imanni, H. S., El Harti, A., El Mostafa, B., Mouncif, H., Eddassouqui, F., Achraf Hasnai, M., & Ismail Zinelabidine, M. (2023). Multispectral UAV data for detection of weeds in a citrus farm using machine learning and Google Earth Engine: Case study of Morocco. Remote Sensing Applications: Society and Environment, 30, 100941. https://doi.org/10.1016/j.rsase.2023.100941
Estrada-Zúñiga, A. C., Cárdenas, J., Víctor Bejar, J., & Ñaupari, J. (2022). Biomass estimation of a high Andean plant community with multispectral images acquired using UAV remote sensing and Multiple Linear Regression, Support Vector Machine and Random Forests models. Scientia Agropecuaria, 13(3), 301-310. https://doi.org/10.17268/sci.agropecu.2022.027
Ezrari, S., Radouane, N., Thiri, A., El Housni, Z., Mokrini, F., et al. (2022). Dry root rot disease, an emerging threat to citrus industry worldwide under climate change: A review. Physiological and Molecular Plant Pathology. 117, 101753. https://doi.org/10.1016/j.pmpp.2021.101753
Freitas, T. A., Proença, C. A., Baldo, T. A., Materón, E. M., Wong, A., Magnani, R. F., & Faria, C. F. (2019). Ultrasensitive immunoassay for detection of Citrus tristeza virus in citrus sample using disposable microfluidic electrochemical device. Talanta, 205, 120110. https://doi.org/10.1016/j.talanta.2019.07.005
Garcia-Ruiz, F., Sankaran, S., Maja, J. M., Lee, W. S., Rasmussen, J., & Ehsani, R. (2013). Comparison of two aerial imaging platforms for identification of Huanglongbing-infected citrus trees. Computers and Electronics in Agriculture, 91, 106-115. https://doi.org/10.1016/j.compag.2012.12.002
Godefroid, M., (2023). Species distribution models predicting climate suitability for the psyllid Trioza erytreae, vector of citrus greening disease. Crop Protection. 168: 106228. https://doi.org/10.1016/j.cropro.2023.106228
Hajeri, S., & Yokomi, R. (2023). Chapter 6 - Citrus tristeza virus. Plant RNA viruses. 117-133. https://doi.org/10.1016/B978-0-323-95339-9.00009-0
Huang, C., Davis, L. S., & Townshend, J. R. G. (2002). An assessment of support vector machines for land cover classification. International Journal of Remote Sensing, 23(4), 725-749. https://doi:10.1080/01431160110040323
Kruse, F. A., Heidebrecht, K. B., Shapiro, A. T., Barloon, P. J., & Goetz, A. F. H. (1993). The Spectral Image Processing System (SIPS) Interactive Visualization and Analysis of Imaging Spectrometer Data. Remote Sensing of Environment, 44, 145-163. https://doi.org/10.1016/0034-4257(93)90013-N
Kumar, A., Lee, W. S., Ehsani, R. J., Albrigo, L. G., Yang, C., & Mangan, R. L. (2012). Citrus greening disease detection using aerial hyperspectral and multispectral imaging techniques. Journal of Applied Remote Sensing, 6, 063542. https://doi:10.1117/1.JRS.6.063542
Lan, Y., Huang, Z., Deng, X., Zhu, Z., Huang, H., et al. (2020). Comparison of machine learning methods for citrus greening detection on UAV multispectral images. Computers and Electronics in Agriculture, 171, 105234. https://doi.org/10.1016/j.compag.2020.105234
Li, H., Lee, W. S., Wang, K., Ehsani, R., & Yang, C. (2013). Extended spectral angle mapping (ESAM) for citrus greening disease detection using airborne hyperspectral imaging. Precision Agriculture, 15(2). https://doi:10.1007/s11119-013-9325-6
Milind, L. (2023). Chapter 23 - World fresh citrus trade and quarantine issues. Citrus Fruit (second Edition). Biology, Technology and Evaluation. 737-761. https://doi.org/10.1016/B978-0-323-99306-7.00017-7
Moriya, É. A. S., Imai, N. N., Tommaselli, A. M. G., Berveglieri, A., Santos, G. H., Soares, M. A., Marino, M., & Reis, T. T. (2021). Detection and mapping of trees infected with citrus gummosis using UAV hyperspectral data. Computers and Electronics in Agriculture, 188, 106298. https://doi.org/10.1016/j.compag.2021.106298
Moussaid, A., El Fkihi, S., & Zennayi, Y. (2020). Citrus orchards monitoring based on remote sensing and artificial intelligence techniques: A review of the literature. Science and In Proceedings of the 2nd International Conference on Advanced Technologies for Humanity - ICATH, 1, 172-178. https://doi.org/10.5220/0010432001720178
Pal, M. (2005). Random forest classifier for remote sensing classification. International Journal of Remote Sensing, 26(1), 217-222. https://doi.org/10.1080/01431160412331269698
Pino-Vargas, E. M., & Huayna, G. (2022). Spatial and temporal evolution of olive cultivation due to pest attack, using remote sensing and satellite image processing. Scientia Agropecuaria, 13(2), 149-157. https://doi.org/10.17268/sci.agropecu.2022.013
Rozenberg, G., Kent, R., & Blank, L. (2021). Consumer‑grade UAV utilized for detecting and analyzing late‑season weed spatial distribution patterns in commercial onion felds. Precision Agriculture, 22, 1317-1332. https://doi.org/10.1007/s11119-021-09786-y
Scheeres, J., De Jong, J., Brede, B., Brancalion, P. H. S., Broadbent, E. N., et al. (2023). Distinguishing forest types in restored tropical landscapes with UAV-borne LIDAR. Remote Sensing of Environment, 290, 113533. https://doi.org/10.1016/j.rse.2023.113533
Shao, G., Han, W., Zhang, H., Liu, S., Wang, Y., Zhang, L., & Cui, X. (2021). Mapping maize crop coefficient Kc using random forest algorithm based on leaf area index and UAV-based multispectral vegetation indices. Agricultural Water Management, 252, 106906. https://doi.org/10.1016/j.agwat.2021.106906
SAGARPA (2021). Sistema de Información Agroalimentaria de Consulta (SIACON). Servicio de Información Agroalimentaria y Pesquera (SIAP). https://www.gob.mx/siap/documentos/siacon-ng-161430. Consulta 3 de abril de 2023
Spreen, T. H., Gao, Z., Fernandes, W., & Zansler, M. L. (2020). Global economics and marketing of citrus products. The Genus Citrus, 471-493. https://doi:10.1016/b978-0-12-812163-4.00023-1
Sun, J., Yang, J., Zhang, C., Yun, W., & Qu, J. (2013). Automatic remotely sensed image classification in a grid environment based on the maximum likelihood method. Mathematical and Computer Modelling, 58(3-4), 573-581. https://doi.org/10.1016/j.mcm.2011.10.063
Tuwo, M., Kuswinanti, T., Nasruddin, A. & Tambaru, E. 2024. Uncovering the presence of CVPD disease in citrus varieties of South Sulawesi, Indonesia: A molecular approach. Journal of Genetic Engineering and Biotechnology, 22, 100332. https://doi.org/10.1016/j.jgeb.2023.100332
Xie, C., & Lee, W. S. (2021). Detection of citrus black spot symptoms using spectral reflectance. Postharvest Biology and Technology, 180, 111627. https://doi.org/10.1016/j.postharvbio.2021.111627
Xu, C., Alhejaili, W., Saifullah, S., Khan, A., Khan, J., & El-Shorbagy, M.A. (2022). Analysis of Huanglongbing disease model with a novel fractional piecewise approach. Chaos, Solitons & Fractals. 161: 112316. https://doi.org/10.1016/j.chaos.2022.112316
Yagoub, H., Belbachir, A. H., & Benabadji, N. (2014). Detection and mapping vegetation cover based on the Spectral Angle Mapper algorithm using NOAA AVHRR data. Advances in Space Research, 53(12), 1686-1693. https://doi.org/10.1016/j.asr.2014.03.020
Zabihi, H., Vogeler, I., Amin, Z. M., & Gourabi, B. R. (2016). Mapping the sensitivity of citrus crops to freeze stress using a geographical information system in Ramsar, Iran. Weather and Climate Extremes, 14, 17-23. https://doi.org/10.1016/j.wace.2016.10.002
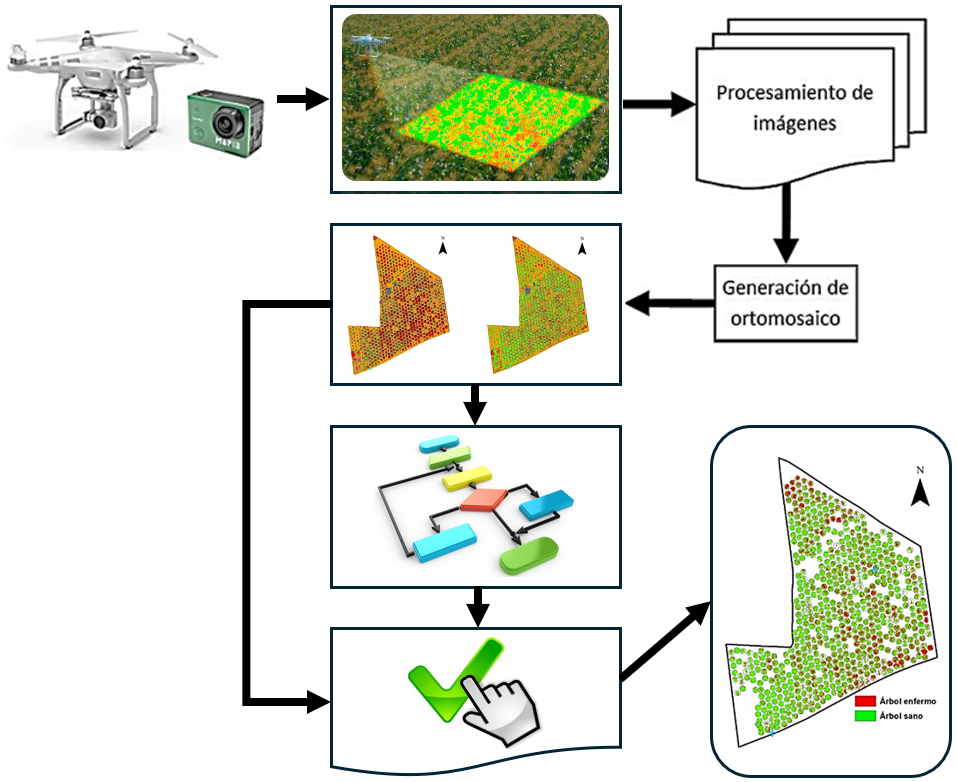
Descargas
Publicado
Cómo citar
Número
Sección
Licencia
Derechos de autor 2024 Scientia Agropecuaria

Esta obra está bajo una licencia internacional Creative Commons Atribución-NoComercial 4.0.
Los autores que publican en esta revista aceptan los siguientes términos:
a. Los autores conservan los derechos de autor y conceden a la revista el derecho publicación, simultáneamente licenciada bajo una licencia de Creative Commons que permite a otros compartir el trabajo, pero citando la publicación inicial en esta revista.
b. Los autores pueden celebrar acuerdos contractuales adicionales separados para la distribución no exclusiva de la versión publicada de la obra de la revista (por ejemplo, publicarla en un repositorio institucional o publicarla en un libro), pero citando la publicación inicial en esta revista.
c. Se permite y anima a los autores a publicar su trabajo en línea (por ejemplo, en repositorios institucionales o en su sitio web) antes y durante el proceso de presentación, ya que puede conducir a intercambios productivos, así como una mayor citación del trabajo publicado (ver efecto del acceso abierto).